How AI and Data-Driven Decisions are Reshaping Executive Leadership
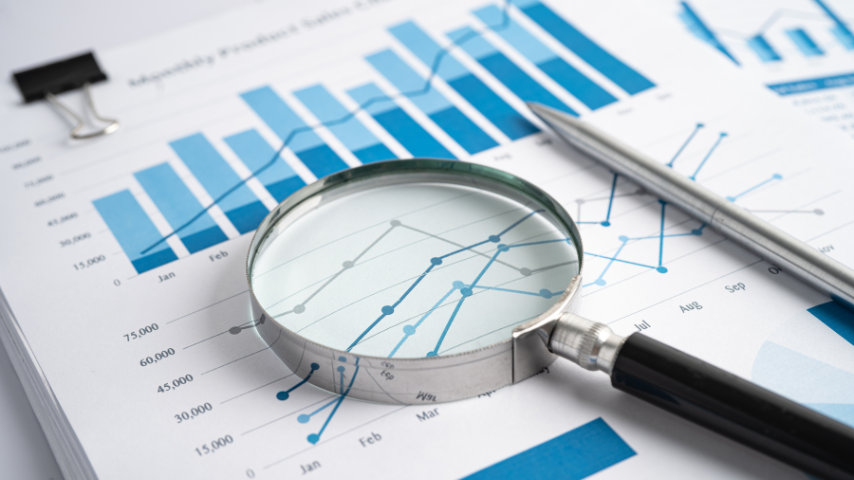
Data-Driven Decision Making: A Guide for Executives
In today's digital age, data is the lifeblood of strategic business decisions. Gone are the days when executive leadership relied solely on intuition, experience, or manual analysis. Now, with the surge in advanced technologies like artificial intelligence (AI), machine learning (ML), and advanced analytics, data-driven decision-making has transformed into a robust framework that offers precision, scalability, and real-time insights. For executives, understanding and harnessing the power of data in decision-making is not just an option—it’s a necessity for maintaining competitive advantage.
This guide explores the core principles of data-driven decision-making (DDDM) while linking it to the latest technologies that can revolutionize your business strategies.
Why Data-Driven Decision Making Matters
Data-driven decision-making refers to the process of making business decisions based on data analysis and interpretation rather than guesswork or anecdotal evidence. For executives, the implications of this shift are profound:
- Improved accuracy: Leveraging data reduces the likelihood of error or oversight, ensuring decisions are rooted in verifiable facts.
- Faster decision-making: Real-time data analysis tools and dashboards provide insights quickly, allowing for agile responses.
- Risk mitigation: By using predictive models, companies can forecast outcomes and proactively manage risks.
Yet, while the benefits of data-driven decision-making are well-established, many organizations still struggle to effectively implement it. The key to overcoming these challenges lies in pairing DDDM with cutting-edge technologies such as AI, advanced analytics, and machine learning.
AI and Machine Learning: Revolutionizing Decision Making
One of the most significant enablers of data-driven decision-making today is AI and machine learning. These technologies allow organizations to analyze vast amounts of structured and unstructured data, identify patterns, and make predictions far more quickly and accurately than human analysts could.
AI-Powered Predictive Analytics
AI enhances decision-making through predictive analytics, which goes beyond simple data analysis by forecasting future trends. For example, machine learning algorithms can sift through massive customer data sets to predict purchasing behavior or identify potential churn risks. By anticipating these trends, executives can make proactive decisions that improve customer retention, product development, or market strategies.
Automation in Robotics
In industries like manufacturing and logistics, robotics, powered by AI and machine learning, uses data to optimize operations. Robots equipped with sensors and AI systems can process real-time data, allowing for immediate adjustments to workflows. This capability translates to more efficient processes, reduced downtime, and improved safety. Executives overseeing these sectors can leverage such data to fine-tune operations and drive continuous improvement.
Enhanced Decision Models with Machine Learning
Machine learning also plays a critical role in refining decision models. Traditionally, decision-making frameworks depended on historical data and static rules. Machine learning, however, enables these models to evolve dynamically. A financial institution, for instance, could use ML to update credit scoring algorithms based on real-time repayment patterns rather than relying solely on traditional metrics. This ensures that decision-making stays current and aligned with ever-changing conditions.
Advanced Analytics: Deepening Insights for Informed Decisions
Beyond AI and machine learning, advanced analytics offer valuable tools for executives seeking to deepen their understanding of complex data. Techniques such as sentiment analysis, prescriptive analytics, and real-time dashboards provide not only descriptive insights but also actionable recommendations.
Descriptive and Diagnostic Analytics
Descriptive analytics helps organizations understand what has happened in the past, using historical data to identify trends. Diagnostic analytics, on the other hand, explains why something occurred by drilling down into root causes. For executives, the combination of these two approaches provides a foundation for strategic decision-making. For example, a company may use diagnostic analytics to investigate why a particular product line underperformed, allowing leadership to adjust marketing or manufacturing strategies accordingly.
Prescriptive Analytics for Optimized Outcomes
Taking this a step further, prescriptive analytics uses AI to suggest the best course of action based on predicted outcomes. Retail executives could use prescriptive models to adjust pricing strategies in real time based on customer purchasing behavior, competitive pricing, and supply chain data. This level of sophistication makes it easier to act on data rather than just observe it.
Real-Time Analytics Dashboards
For executives, staying on top of fast-moving data is crucial. Real-time analytics dashboards offer a consolidated view of key metrics and trends, updated instantaneously. These dashboards, often powered by AI and machine learning, can alert leaders to changes in KPIs, market shifts, or operational bottlenecks. By visualizing this data, executives can make quicker, better-informed decisions and respond immediately to potential disruptions.
The Role of Data Culture in Executive Decision-Making
While the tools and technologies are essential, the most critical element of successful data-driven decision-making is fostering a data-centric culture. Executives must champion a data-first mindset within their organizations. This means:
- Encouraging data literacy: Executives should invest in training and tools that make data accessible and understandable for employees across all departments.
- Promoting data transparency: Leaders should emphasize the importance of transparency, ensuring that decisions are traceable and backed by clear data.
- Building cross-functional teams: Collaboration between IT, data scientists, and business leaders is crucial for aligning data with business objectives.
Overcoming Common Challenges
Despite its transformative potential, DDDM is not without its challenges. Data silos, lack of integration, and inadequate infrastructure are common barriers that prevent organizations from fully capitalizing on their data. To overcome these obstacles, executives should prioritize:
- Data integration strategies: Implementing cloud-based data warehouses or lakes that consolidate data from across the organization.
- Scalable infrastructure: Leveraging platforms with robust computational power, such as AI-driven analytics platforms, to handle big data efficiently.
- Ethical considerations: Ensuring data privacy and security remain top priorities when making data-driven decisions, especially when leveraging AI or machine learning models.
Conclusion: A New Era of Executive Decision-Making
Data-driven decision-making is no longer a choice but an imperative for executives in today’s technology-driven world. By harnessing the power of AI, machine learning, and advanced analytics, executives can unlock new opportunities for growth, optimize operations, and reduce risks.
As technology continues to evolve, the future of decision-making will likely see even deeper integration of robotics, AI, and real-time analytics. Executives who embrace these tools now will be better positioned to lead their organizations into the next era of business intelligence, driving success in an increasingly complex and competitive landscape.