Can Machine Learning Detect Alzheimer’s Early?
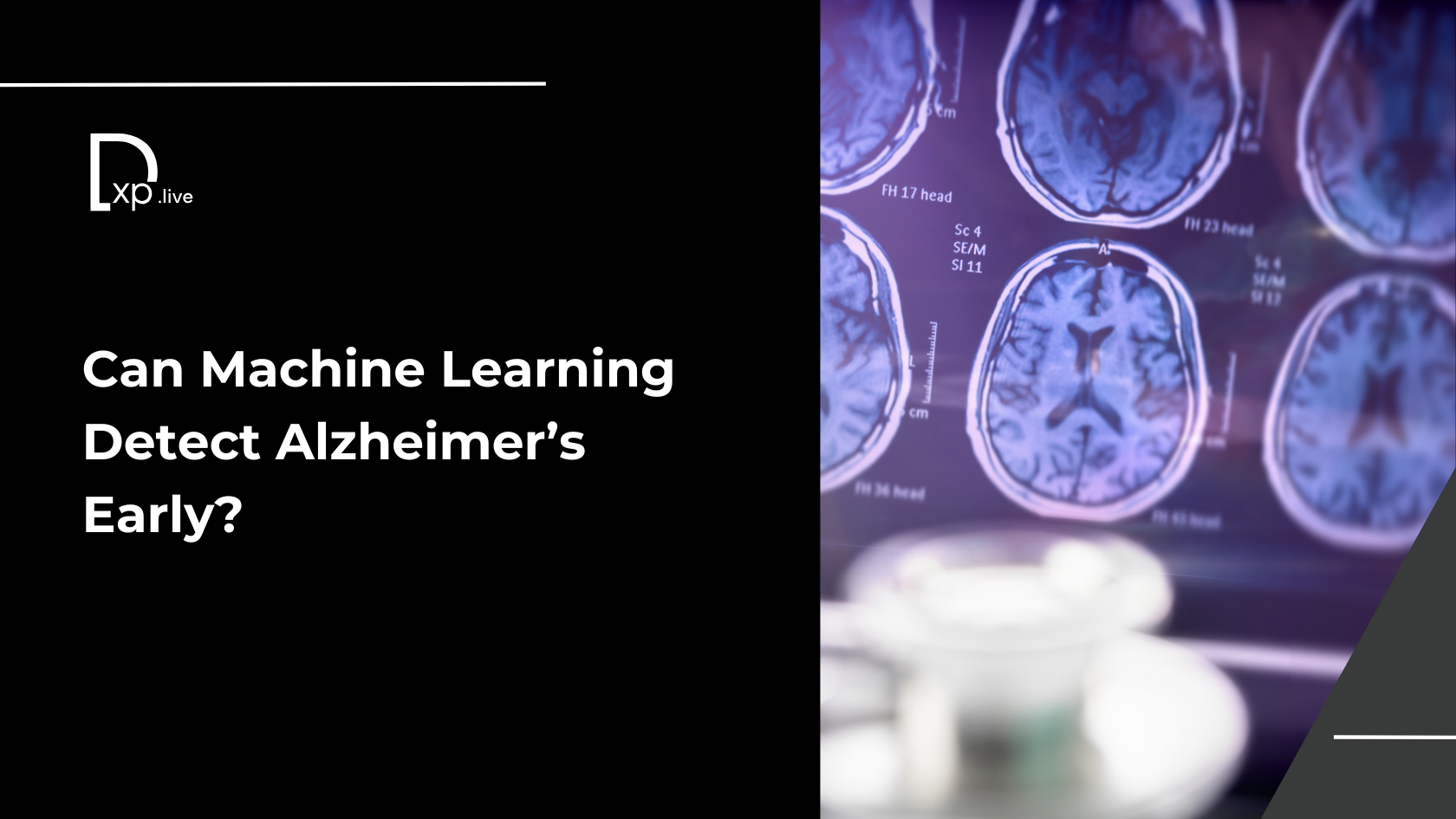
Machine learning, a subset of artificial intelligence, has taken center stage in revolutionizing industries worldwide. In medicine, its transformative potential is reshaping how diseases are diagnosed, monitored, and treated. A recent breakthrough—a video-based machine learning tool capable of identifying early signs of Alzheimer’s disease—exemplifies how this technology is pushing the boundaries of traditional healthcare.
This tool, Variational Animal Motion Embedding (VAME), developed by Gladstone Institutes, analyzed subtle behavioral changes in mice, offering new insights into Alzheimer’s progression. By detecting disorganized behavior long before conventional tests could, this innovation underscores how machine learning is setting new standards in early diagnosis and treatment evaluation.
How Machine Learning is Redefining Disease Diagnosis
Machine learning (ML) is an integral part of modern medicine. Its applications range from analyzing genetic data and imaging to predicting patient outcomes and personalizing treatment plans. By processing vast amounts of data, identifying patterns, and making predictions with precision, machine learning is bridging gaps in human expertise and time-intensive processes.
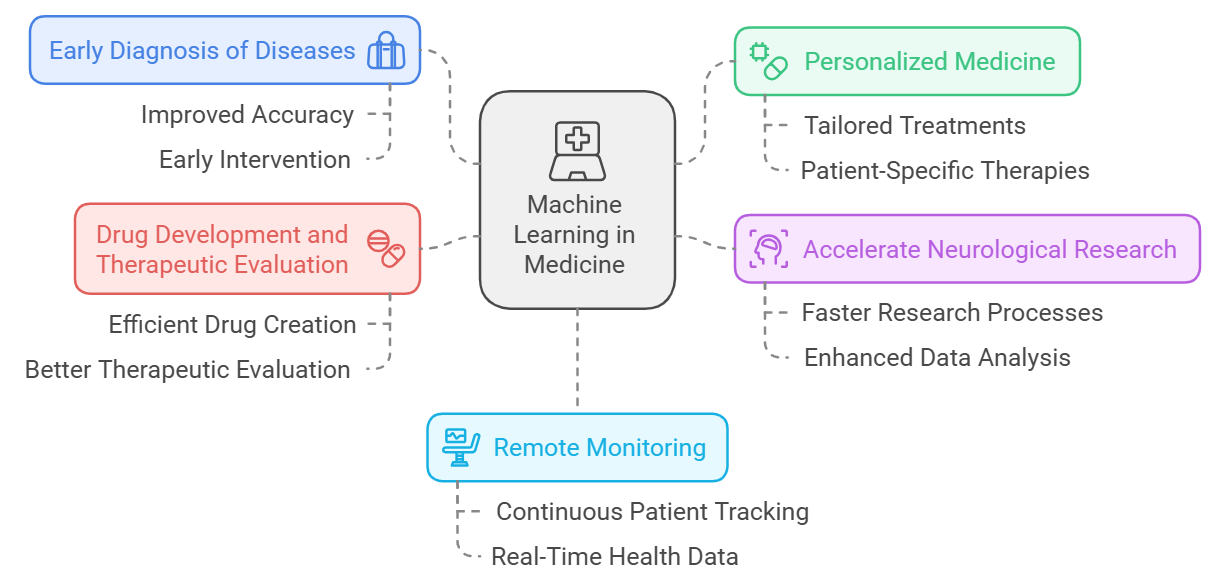
1. Early Diagnosis of Diseases
One of the most significant impacts of machine learning is its ability to detect diseases in their early stages. In the case of Alzheimer’s, early behavioral changes often go unnoticed, making timely intervention a challenge. The VAME tool’s ability to analyze video footage and detect subtle signs of disorganized behavior demonstrates the unmatched potential of machine learning in early diagnosis.
Beyond Alzheimer’s, ML models are being used in cancer detection, cardiovascular disease prediction, and diabetes monitoring. For instance, radiology has seen a paradigm shift, with ML algorithms analyzing medical imaging data faster and more accurately than traditional methods. Tools like Google’s AI for breast cancer screening are reducing false positives and negatives, saving lives by enabling timely treatment.
2. Personalized Medicine
Machine learning enables healthcare providers to move away from one-size-fits-all approaches. By analyzing genetic, environmental, and lifestyle factors, ML algorithms can recommend personalized treatment plans tailored to an individual’s unique needs. In oncology, for example, machine learning helps determine the most effective chemotherapy regimen for a patient based on tumor characteristics and genetic profiles.
This shift toward precision medicine ensures better outcomes, fewer side effects, and more efficient use of healthcare resources. With tools like VAME, personalizing interventions for neurological diseases could become a reality, paving the way for more targeted therapies.
3. Revolutionizing Neurological Research
Neurological disorders, such as Alzheimer’s, Parkinson’s, and epilepsy, are notoriously complex and often progress silently. Traditional methods of studying these diseases involve labor-intensive behavioral tests with limited scalability. Machine learning offers a game-changing solution by analyzing spontaneous behaviors through non-invasive methods like video analysis.
In the study published by Gladstone Institutes, VAME detected increased transitions between activities in mice, a subtle marker of cognitive decline. This insight provides researchers with valuable data, enabling them to explore disease mechanisms more effectively. Machine learning tools like VAME are poised to accelerate neurological research by providing scalable, unbiased methods to track disease progression.
4. Drug Development and Therapeutic Evaluation
Drug discovery and therapeutic evaluation have traditionally been lengthy, costly processes. Machine learning is streamlining these stages by analyzing biological data, predicting drug efficacy, and identifying potential side effects before clinical trials. In the case of Alzheimer’s, Gladstone researchers used VAME to test the effectiveness of blocking fibrin-induced inflammation. The tool’s unbiased assessment of behavior changes in treated mice validated the therapeutic strategy, reaffirming machine learning’s value in preclinical studies.
5. Remote Monitoring and Accessibility
Machine learning tools are making healthcare more accessible. With technologies like VAME capable of analyzing smartphone-quality video, patients can be monitored in their homes. This reduces the need for frequent clinic visits and enables continuous observation, which is particularly valuable for patients with chronic or progressive diseases. By identifying patterns in everyday behavior, these tools can alert caregivers and physicians to early warning signs, ensuring timely interventions.
Transformative Potential for the Future
The integration of machine learning into medicine is still in its early stages, but its potential is boundless.
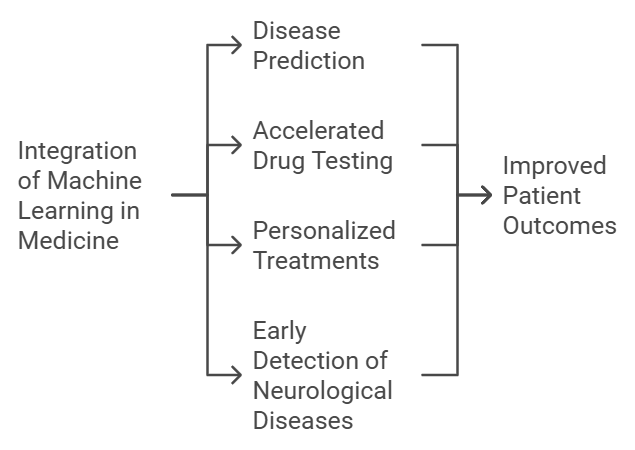
Imagine a world where:
- Doctors can predict a disease before symptoms arise.
- Researchers can test new drugs faster and more accurately.
- Patients can receive treatments tailored specifically to their genetic and environmental profiles.
- Neurological diseases, often diagnosed too late, can be detected decades earlier.
These possibilities are becoming realities as machine learning continues to evolve. However, challenges remain, including ensuring data privacy, reducing algorithmic biases, and improving interpretability. Collaborative efforts between scientists, clinicians, and technologists will be critical in addressing these issues and unlocking machine learning’s full potential.
Future of Healthcare
Machine learning is reshaping medicine in unprecedented ways, as demonstrated by its application in early Alzheimer’s detection through tools like VAME. By enabling early diagnosis, personalizing treatments, accelerating drug development, and making healthcare more accessible, ML is breaking barriers and setting new benchmarks.
As technology advances, machine learning will continue to revolutionize how we understand and combat diseases. From neurological disorders to oncology and beyond, its impact is profound and far-reaching.
Subscribe to our newsletter to stay updated on the latest advancements, expert insights, and thought-provoking discussions in the world of machine learning and beyond. Join us in exploring how technology is shaping the future of medicine!
FAQs
1. How is machine learning used in medical diagnosis?
Machine learning is used to analyze complex medical data, such as imaging scans, genetic information, and patient histories, to detect diseases early, predict outcomes, and personalize treatments.
2. What diseases can machine learning help diagnose?
Machine learning is applied in diagnosing various conditions, including Alzheimer’s, cancer, cardiovascular diseases, diabetes, and rare genetic disorders, often at early stages.
3. How does machine learning improve early disease detection?
By identifying subtle patterns and anomalies in data that might be missed by human observation, machine learning detects diseases in their earliest stages.
4. What are the challenges of using machine learning in healthcare?
Challenges include ensuring data privacy, overcoming biases in algorithms, gaining regulatory approval, and integrating ML tools into existing healthcare systems.
5. Can machine learning replace doctors in diagnosis?
Machine learning complements doctors by providing faster, data-driven insights, but it cannot replace the expertise and judgment of medical professionals.