What is the difference between Machine Learning and Artificial Intelligence?
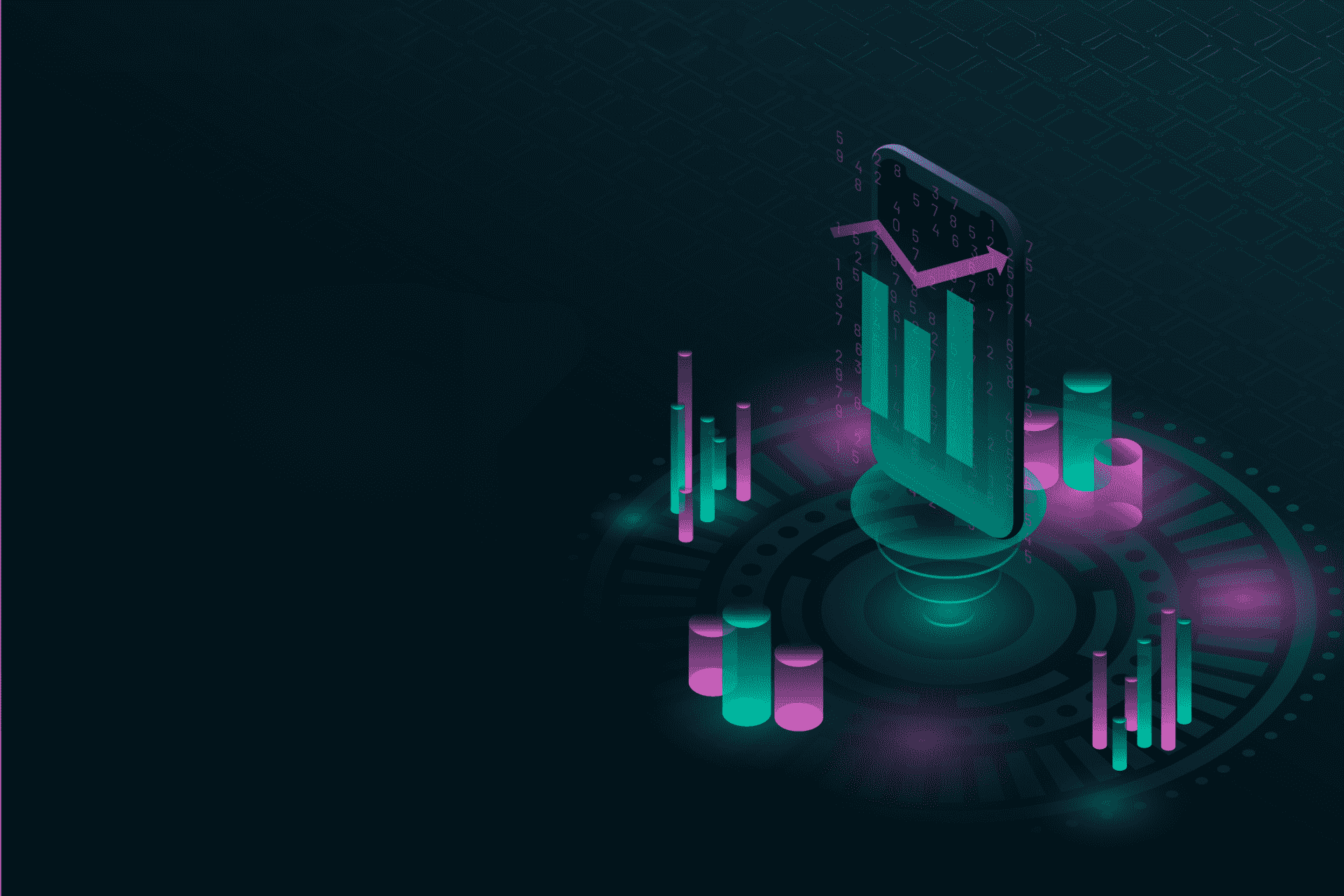
Machine Learning vs. Artificial Intelligence: What Business Leaders Need to Know in the Age of LLMs
Today's business leaders are increasingly confronted with terms like Machine Learning (ML) and Artificial Intelligence (AI). While these concepts are often used interchangeably, understanding their distinctions and implications is crucial for C-suite executives aiming to leverage these technologies for business growth and innovation. This article will delve into the differences between ML and AI, their business applications, and their role in the context of Large Language Models (LLMs) like ChatGPT, Claude, and Gemini.
Defining AI and ML
Artificial Intelligence, in its broadest sense, refers to the development of computer systems capable of performing tasks that typically require human intelligence. These tasks include visual perception, speech recognition, decision-making, and language translation. AI aims to create machines that can mimic or surpass human cognitive functions.
Machine Learning, on the other hand, is a subset of AI. It focuses on the development of algorithms and statistical models that enable computer systems to improve their performance on a specific task through experience, without being explicitly programmed. In essence, ML allows systems to learn from data and make predictions or decisions without human intervention.
Key Differences
- Scope: AI is a wider concept encompassing various approaches to creating intelligent systems, while ML is a specific method within AI that focuses on data-driven learning.
- Functionality: AI systems can be designed to handle a broad range of tasks, while ML models are typically specialized for specific functions or problem domains.
- Human intervention: Traditional AI systems often rely on pre-programmed rules and logic, while ML systems learn patterns from data with minimal human guidance.
- Adaptability: ML systems can adapt and improve their performance over time as they process more data, whereas traditional AI systems may require manual updates to their rule sets.
Business Applications
Both AI and ML offer significant potential for businesses across various industries. Here are some key applications:
- Customer Service: AI-powered chatbots and virtual assistants can handle customer inquiries 24/7, improving response times and customer satisfaction.
- Predictive Analytics: ML algorithms can analyze historical data to forecast trends, customer behavior, and market conditions, enabling more informed decision-making.
- Fraud Detection: AI and ML systems can identify unusual patterns in financial transactions, helping to prevent fraud and reduce financial risks.
- Supply Chain Optimization: ML models can analyze supply chain data to optimize inventory management, predict demand, and improve logistics efficiency.
- Personalization: AI-driven recommendation systems can enhance user experiences in e-commerce, content streaming, and digital marketing.
- Process Automation: AI and ML can automate repetitive tasks, increasing operational efficiency and allowing employees to focus on higher-value activities.
The Rise of Large Language Models (LLMs)
In recent years, the development of Large Language Models has revolutionized the field of AI and ML. LLMs are advanced AI systems trained on vast amounts of text data, enabling them to understand and generate human-like text across a wide range of topics and tasks. Notable examples include OpenAI's ChatGPT, Anthropic's Claude, and Google's Gemini.
LLMs represent a convergence of AI and ML technologies, showcasing how machine learning techniques can be applied to create more general-purpose AI systems. These models leverage deep learning architectures, particularly transformer models, to process and generate text with unprecedented fluency and contextual understanding.
Business Implications of LLMs
For C-suite executives, understanding the potential of LLMs is crucial in today's competitive landscape. Here are some key considerations:
- Enhanced Productivity: LLMs can assist employees in various tasks, from drafting emails and reports to analyzing complex data sets, potentially boosting productivity across the organization.
- Improved Decision-Making: By processing and synthesizing vast amounts of information, LLMs can provide executives with valuable insights and summaries to support strategic decision-making.
- Innovation in Customer Interaction: LLMs can power more sophisticated chatbots and virtual assistants, enabling more natural and context-aware customer interactions.
- Content Creation and Marketing: These models can assist in generating marketing copy, product descriptions, and personalized content at scale, potentially revolutionizing content marketing strategies.
- Research and Development: LLMs can accelerate R&D processes by quickly summarizing research papers, generating hypotheses, and even assisting in code generation for software development.
- Language Translation and Localization: Multi-lingual LLMs can facilitate global business expansion by providing more accurate and context-aware translations for international markets.
Challenges and Considerations
While the potential of AI, ML, and LLMs is immense, business leaders must also be aware of the challenges:
- Data Privacy and Security: The use of AI and ML often involves processing large amounts of data, raising concerns about data privacy and security. Ensuring compliance with regulations like GDPR and CCPA is crucial.
- Ethical Considerations: As AI systems become more advanced, questions about bias, fairness, and transparency in decision-making processes become increasingly important.
- Integration and Implementation: Incorporating AI and ML solutions into existing business processes can be complex and may require significant investment in infrastructure and training.
- Skill Gap: There is a growing demand for AI and ML expertise, and organizations may face challenges in recruiting and retaining skilled professionals.
- Reliability and Accountability: As businesses rely more on AI-driven decisions, establishing clear accountability frameworks and ensuring the reliability of AI systems becomes critical.
- Rapid Technological Evolution: The field of AI and ML is evolving rapidly, and staying current with the latest advancements can be challenging for businesses.
Future Outlook
As AI and ML technologies continue to advance, we can expect to see:
- More Specialized AI Applications: While general-purpose AI like LLMs will continue to evolve, we'll likely see an increase in specialized AI solutions tailored for specific industries and use cases.
- Enhanced Human-AI Collaboration: The focus will shift towards developing AI systems that augment human capabilities rather than replace them, fostering more effective human-AI collaboration in the workplace.
- Democratization of AI: As AI tools become more accessible, smaller businesses will increasingly be able to leverage these technologies, potentially leveling the playing field with larger corporations.
- Regulatory Developments: We can expect more comprehensive regulations governing the development and use of AI, particularly in high-stakes applications like healthcare and finance.
- Advancements in Explainable AI: As AI systems become more complex, there will be a growing emphasis on developing techniques to make AI decision-making processes more transparent and interpretable.
Conclusion
For C-suite executives, understanding the distinctions between AI and ML, as well as their evolving applications in the form of LLMs, is no longer optional—it's a strategic imperative. These technologies offer unprecedented opportunities for innovation, efficiency, and competitive advantage. However, they also present challenges that require careful consideration and planning.
As we move further into the age of AI, successful business leaders will be those who can effectively navigate this complex landscape, balancing the potential of these technologies with ethical considerations and strategic implementation. By staying informed and adaptable, executives can position their organizations to thrive in an increasingly AI-driven business world.