Edge Analytics: Revolutionizing Real-Time Data Processing
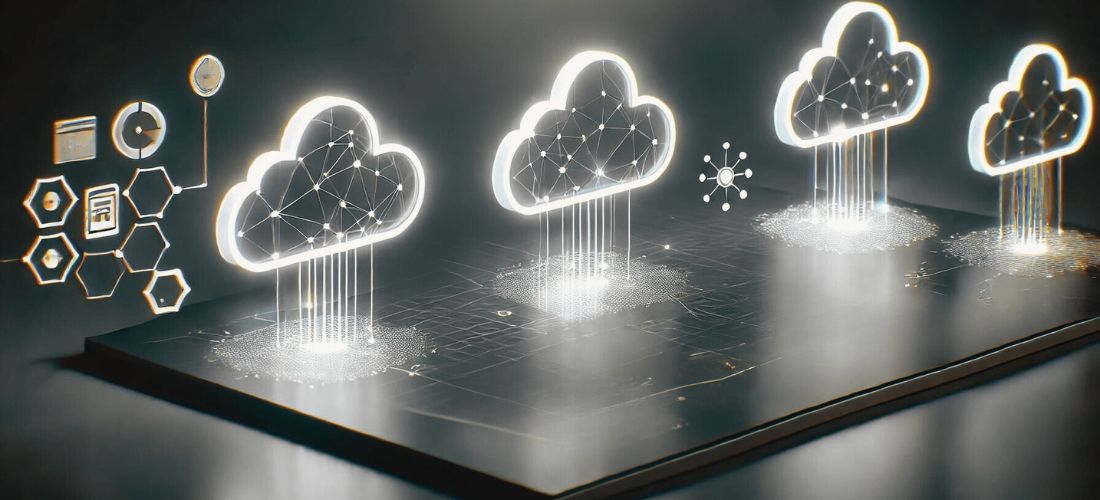
As digital transformation advances, real-time data processing has become crucial for organizational success. Edge analytics is an innovative solution that brings data analysis closer to the data source. By leveraging edge analytics, organizations can achieve faster, more informed decision-making. This blog explores what edge analytics is, how it works, its benefits, and its applications.
What is Edge Analytics
Edge analytics involves analyzing data at the network's edge, where it is generated, rather than sending it to a centralized data center. IoT sensors, industrial machines, and surveillance equipment process and analyze data locally. This local processing reduces latency, conserves bandwidth, and enables quicker, more efficient decision-making.
How Edge Analytics Works
Edge analytics utilizes a decentralized approach distinct from traditional data analytics. Here’s a breakdown of its process:
- Data Collection: Edge devices and sensors gather raw data from various sources.
- Local Pre-processing: The data is filtered, cleaned, and compressed locally, reducing volume.
- On-site Analysis: Local algorithms and models analyze the pre-processed data.
- Data Transmission: Only critical data or significant findings are sent to a central data center for further analysis or storage.
Handling data close to its source significantly improves the speed and efficiency of data processing and analysis.
Benefits of Edge Analytics
The advantages of edge analytics extend beyond just faster data processing. Key benefits include:
- Accelerated Decision-making: Processing data at the edge reduces the time between data collection and analysis, enabling real-time insights and faster decision-making.
- Reduced Latency: Minimizes the delays associated with transmitting data to a remote processing center, which is crucial for applications like IoT and predictive maintenance.
- Lower Bandwidth Requirements: By handling data analysis locally, edge analytics minimizes the volume of data that must be transmitted to central servers. This approach conserves network bandwidth and reduces associated transmission costs .
- Enhanced Data Security and Privacy: Local processing of sensitive data minimizes the risk of data breaches during transmission.
- Scalability: Supports the growth of IoT devices by distributing data processing across multiple nodes, allowing organizations to handle increasing data volumes without overloading the network or central systems.
Applications of Edge Analytics
Edge analytics can be applied across various industries, each benefiting uniquely from this technology:
- Agriculture: In precision farming, edge analytics monitors soil conditions, weather, and crop health in real-time. Field sensors collect data on soil moisture, temperature, and nutrient levels, which are analyzed on-site to optimize irrigation and fertilization schedules.
- Energy Management: Used to monitor and manage renewable energy sources like solar panels and wind turbines. By analyzing data locally, energy providers can predict equipment failures, optimize energy production, and balance supply and demand in real time.
- Public Safety: Enhances public safety by processing data from surveillance cameras and sensors in real time, detecting unusual activities, and alerting authorities immediately.
- Environmental Monitoring: Applied in monitoring ecosystems and wildlife by analyzing data on air and water quality, animal movements, and vegetation health in real time.
- Telecommunications: Improves customer service by managing network performance through local data analysis from cell towers and network nodes.
- Oil and Gas Industry: Processes sensor data monitoring equipment performance and environmental conditions in offshore oil rigs, predicting equipment failures, and optimizing maintenance schedules.
- Hospitality: Enhances guest experiences by analyzing data from smart devices in guest rooms and personalizing services like room temperature, lighting, and entertainment options.
- Supply Chain Management: Tracks the condition and location of goods in transit by analyzing sensor data in shipping containers, ensuring perishable goods remain within safe conditions.
Practical Solutions for Implementing Edge Analytics
Implementing edge analytics requires strategic planning and the right resources. Here are some practical steps:
- Selecting Appropriate Hardware and Software: Choose devices and platforms that can handle the processing power and storage requirements of your edge analytics applications.
- Developing and Deploying Algorithms: Develop and implement analytical models specifically designed for your requirements. Train machine learning models using historical data and adjust them for real-time analysis at the edge.
- Ensuring Security and Compliance: Implement robust security measures to protect data at the edge, ensuring data encryption, secure communication protocols, and compliance with relevant regulations and industry standards.
- Starting Small and Scaling Up: Begin with a pilot project to test the edge analytics implementation, allowing organizations to identify potential issues and refine their approach before scaling up.
- Collaborating with Experts: Partner with vendors or consultants with expertise in edge analytics to navigate the intricacies of deployment.
- Investing in Talent Development: Ensure your organization has skilled personnel to support edge analytics initiatives, including data scientists, engineers, and IT professionals.
- Continuous Monitoring and Optimization: Regularly review analytics models, update them based on new data, and optimize systems to ensure peak performance.
Challenges and Considerations
While edge analytics offers numerous benefits, it also presents some challenges:
- Hardware Limitations: Not all edge devices have the processing power or storage capacity required for complex data analysis.
- Data Quality and Management: Ensuring the accuracy and quality of data collected at the edge is crucial for reliable analysis.
- Integration with Central Systems: Seamlessly integrating edge analytics with existing centralized data systems can be challenging.
Transform Your Operations with Edge Analytics
Edge analytics revolutionizes data processing by delivering significant enhancements in speed, efficiency, and scalability. By processing data at the edge, organizations can make real-time decisions, reduce latency, and improve data security. As the digital landscape evolves, edge analytics will play a major role in driving innovation and enabling smarter, more responsive business operations. Embracing edge analytics today will position your organization for success in the data-driven future.
Read more about the latest trends, success stories, and implementation strategies. Stay informed and ahead of the curve by exploring our in-depth articles on various innovative solutions.