Data Requirements for Training Generative AI Models: A Practical Guide
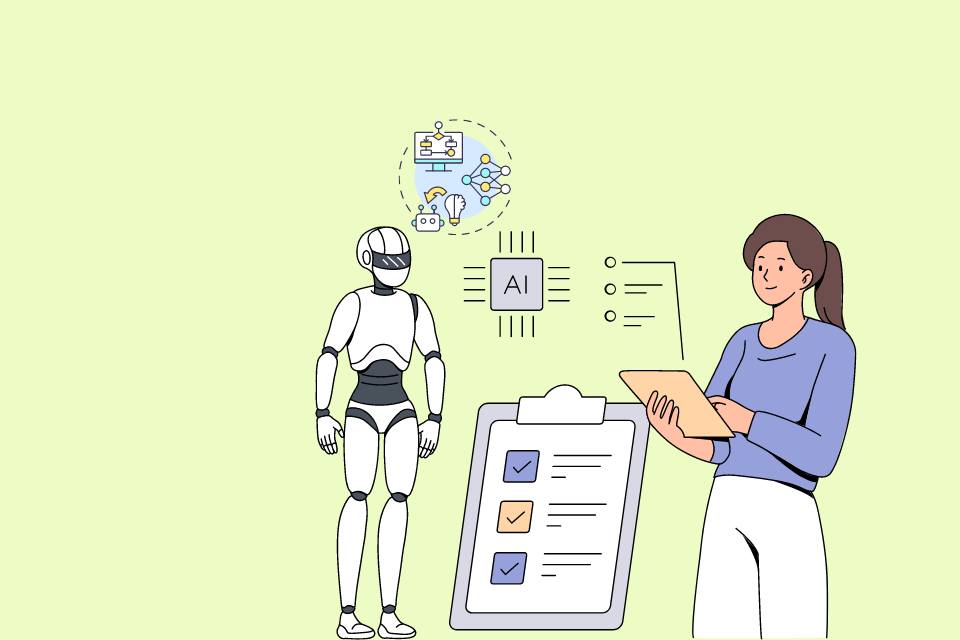
The global market for generative AI reached an estimated $7.5 billion in 2023, reflecting a significant surge in investment and adoption by enterprises seeking to leverage AI for competitive advantage. As companies increasingly turn to generative AI to automate processes, enhance creativity, and drive business innovation, understanding the data requirements for training these models becomes crucial. This blog will outline the critical data considerations for C-suite executives looking to implement generative AI solutions effectively.
Importance of High-Quality Data
Quality data is the cornerstone of any successful AI project. According to Gartner, poor data quality costs organizations an average of $12.9 million annually. For generative AI, the stakes are even higher as the model’s output directly depends on the quality and diversity of the training data. High-quality data ensures that the AI model can generate realistic and relevant outputs, reducing the risk of errors and biases that could lead to costly mistakes or reputational damage.
Key Metrics for Data Quality
- Accuracy: Ensuring that data is correct and free from errors.
- Completeness: Having all necessary data points without missing values.
- Consistency: Maintaining uniformity across different data sources.
- Timeliness: Using up-to-date data to reflect current trends and behaviors.
- Relevance: Ensuring that the data is pertinent to the model's objectives.
Volume and Diversity of Data
Generative AI models, such as GPT-4, require vast data to learn patterns and produce meaningful outputs. McKinsey reports that companies utilizing diverse datasets see a 20-30% improvement in AI model performance. Diversity in data includes not only different types of data (text, images, audio) but also varied sources and contexts.
Data Volume Considerations
- Scalability: The ability to scale data storage and processing capabilities as data volume grows.
- Storage Solutions: Utilizing cloud-based storage solutions like AWS S3 or Google Cloud Storage to handle large datasets efficiently.
- Data Processing: Implementing robust data processing pipelines to handle, clean, and preprocess vast amounts of data before feeding it into AI models.
Ethical and Legal Considerations
As the use of generative AI grows, so do concerns around ethical and legal implications. According to HCL Technologies, 56% of businesses have faced legal challenges related to data privacy and AI ethics. Ensuring compliance with regulations like GDPR, CCPA, and industry-specific guidelines is crucial for avoiding legal repercussions and maintaining public trust.
- Data Privacy: Setting policies to safeguard personal information and maintain adherence to data protection regulations.
- Bias Mitigation: Identifying and mitigating biases in training data to produce fair and unbiased AI models.
- Transparency: Maintaining transparency in data collection and AI model training processes to build trust with stakeholders.
Data Preprocessing and Annotation
Effective data preprocessing and annotation are essential for training high-performing generative AI models. Proper preprocessing ensures that the data is in a suitable format for the AI model, while annotation adds context and meaning to the data.
Steps in Data Preprocessing
- Data Cleaning: Removing duplicates, correcting errors, and filling in missing values.
- Normalization: Scaling data to a standard range to ensure consistent input for the AI model.
- Feature Engineering: Creating new features from raw data to enhance model performance.
Importance of Data Annotation
Data annotation involves labeling data to provide context, which is particularly important for supervised learning models. According to Markets & Markets, the data annotation tools market is expected to reach $2.57 billion by 2027, driven by the increasing demand for high-quality annotated data.
Building a Robust Data Infrastructure
To support the data requirements for generative AI, organizations need a robust data infrastructure. This includes data storage, processing, and management systems that can handle the complexities of large-scale data operations.
Components of a Data Infrastructure
- Data Lakes: Centralized repositories that enable large-scale storage of both structured and unstructured data.
- ETL Pipelines: Extract, Transform, and Load (ETL) pipelines to transmit and manage data effectively.
- Data Governance: Policies and procedures to ensure data quality, security, and compliance.
Way Ahead: Strategic Steps for C-Suite Executives
Generative AI represents a transformative business opportunity, but realizing its potential requires strategic planning and execution. Here are some actionable steps for C-suite executives to ensure successful implementation:
- Invest in Data Quality Initiatives: Prioritize data quality by investing in tools and processes that enhance data accuracy, completeness, and relevance. Establish data quality metrics and regularly monitor them to ensure ongoing improvements.
- Foster a Culture of Data Governance: Develop a robust data governance framework that includes clear data collection, storage, and usage policies. Ensure compliance with legal and ethical standards to protect against risks and build trust with stakeholders.
- Leverage Advanced Data Processing Technologies: Utilize advanced data processing technologies such as AI-driven data cleaning tools and automated ETL pipelines to handle large datasets efficiently. This will guarantee that the best quality data is employed to train your generative AI models.
- Collaborate with Data Experts: Partner with data scientists, engineers, and domain experts to build a multidisciplinary team capable of addressing the complex data requirements of generative AI. Their expertise will be invaluable in navigating challenges and optimizing model performance.
- Continuously Monitor and Update AI Models: Implement a system for continuous monitoring and updating of AI models to ensure they remain relevant and effective. Rewire models frequently utilize fresh data to recognize shifting trends and behaviors.
Are you ready to harness the power of generative AI to transform your business? Explore our blogs on Navigating the Generative AI Revolution: A Guide for Leaders and Generative AI: Reshaping Business Decision-Making for more insights.
By focusing on data quality, governance, and strategic investments, C-suite executives can unlock the full potential of generative AI. Partner with us to navigate the complexities of AI data requirements and drive innovation in your organization.