Real-Time Analytics: Technologies and Implementation
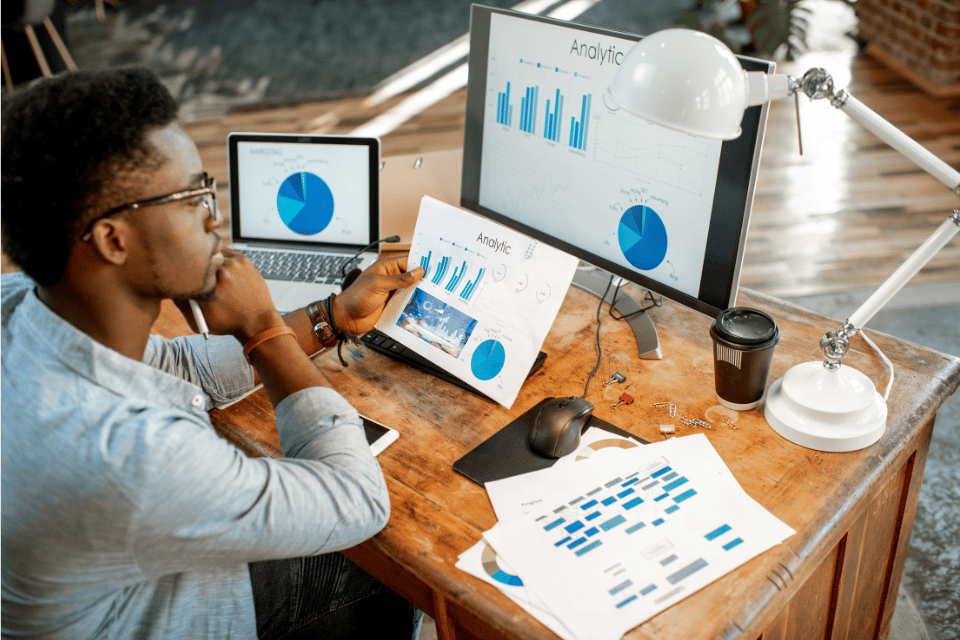
Every second brings new data, real-time analytics has transcended its role as a mere technological upgrade to become a fundamental element of strategic business planning. According to a 2023 survey by IDC, over 70% of large enterprises believe real-time analytics are crucial for making informed decisions and improving operational efficiency. As we navigate through 2024, the ability to harness instantaneous data and convert it into actionable insights is distinguishing industry leaders from the rest.
This detailed exploration delves into the advanced technologies that fuel real-time analytics and offers a clear, actionable guide for C-suite executives. The aim is to equip decision-makers with the necessary tools to seamlessly integrate these capabilities, ensuring their organizations not only adapt but excel in the face of continuous digital innovation.
Key Technologies Driving Real-Time Analytics
- In-Memory Computing: In-memory computing has revolutionized data processing by storing data in RAM instead of traditional databases, which significantly reduces the latency in data retrieval and analysis. For instance, SAP HANA reports that their in-memory computing solutions have helped businesses accelerate analytics processes by up to 1000x.
- Stream Processing Software: Stream processing is essential for analyzing and acting on real-time data streams. Apache Kafka and Apache Storm are leaders in this space, providing frameworks that allow for the processing of data in real-time. These technologies enable businesses to monitor and respond to events as they occur, such as adjusting supply chains instantaneously based on live sales data.
- AI and Machine Learning: AI and machine learning algorithms are increasingly integrated with real-time analytics to predict trends and automate decision-making processes. Tools like TensorFlow and Google Cloud AI provide platforms that analyze large streams of data, learning and adapting in real time. For example, financial institutions leverage these tools to detect fraudulent transactions within milliseconds of their occurrence.
Implementing Real-Time Analytics: A Step-by-Step Guide
Data Integration and Management:
The first step in harnessing real-time analytics is integrating diverse data sources. This involves setting up data pipelines that can handle high-velocity data without bottlenecks. Establishing a seamless data ecosystem is crucial for leveraging real-time analytics effectively. Robust integration frameworks, such as Apache NiFi or Talend, support real-time data ingestion from a myriad of sources, ensuring that data lakes are refreshed instantaneously.
For instance, major financial institutions integrate transactional data across global markets into centralized systems that analyze trends and risks in real-time, enabling proactive responses to market shifts. For example, companies like Netflix use real-time data pipelines to analyze user interactions and optimize streaming quality instantaneously.
Choosing the Right Platform:
Selecting the appropriate platform for real-time analytics depends on the specific needs of the business. The choice of platform should align with the company’s operational requirements and IT infrastructure. While Apache Kafka is excellent for message brokering, Redis is preferred for its low-latency data processing capabilities.
Redis excels in scenarios requiring rapid access to data, such as in high-frequency trading environments where milliseconds can dictate market positions. Conversely, Apache Kafka is suited for scenarios involving vast data streams from multiple sources, such as IoT devices across a manufacturing network, facilitating comprehensive monitoring and operational analytics. The right platform choice is instrumental in optimizing the utility of real-time data and enhancing business responsiveness.
Scaling Infrastructure:
As real-time data analytics demand grows, the underlying infrastructure must scale accordingly. Scalability in infrastructure is a necessity to manage the expanding volumes of data and complexity of analytics. This elasticity is crucial for maintaining performance and managing costs effectively.
Cloud solutions like Microsoft Azure and Amazon Web Services not only provide scalable architecture but also offer advanced services like automated scaling and load balancing and can dynamically adjust resources based on data processing needs. This adaptability is essential for retail giants like Amazon, which experiences variable loads and must scale its operations during peak times like Black Friday sales without service disruption.
Security and Compliance:
In real-time data ecosystems, maintaining stringent security protocols is non-negotiable. Technologies like blockchain and advanced encryption methods (AES) ensure data integrity and security.
For example, healthcare providers utilize blockchain to securely update and share patient records in real-time, maintaining confidentiality and integrity. Compliance is equally critical, with continuous monitoring and updates to practices ensuring adherence to international standards like GDPR in Europe and CCPA in California. This proactive approach is vital for maintaining customer trust and legality in data handling, especially for global enterprises operating across diverse regulatory landscapes.
Harnessing Real-Time Analytics for Future Success
Looking to the future, the integration of IoT devices and edge computing will further enhance the capabilities of real-time analytics. These technologies will bring data processing closer to the data source, reducing latency and increasing the efficiency of data insights. For businesses to remain competitive, adopting a proactive approach to real-time analytics is not just beneficial—it’s imperative.
Follow our website for the insights on latest technologies. Embrace the future of data and unlock new opportunities for business growth and success.