Threat Intelligence: Staying Ahead of Cyber Attacks with Advanced Analytics
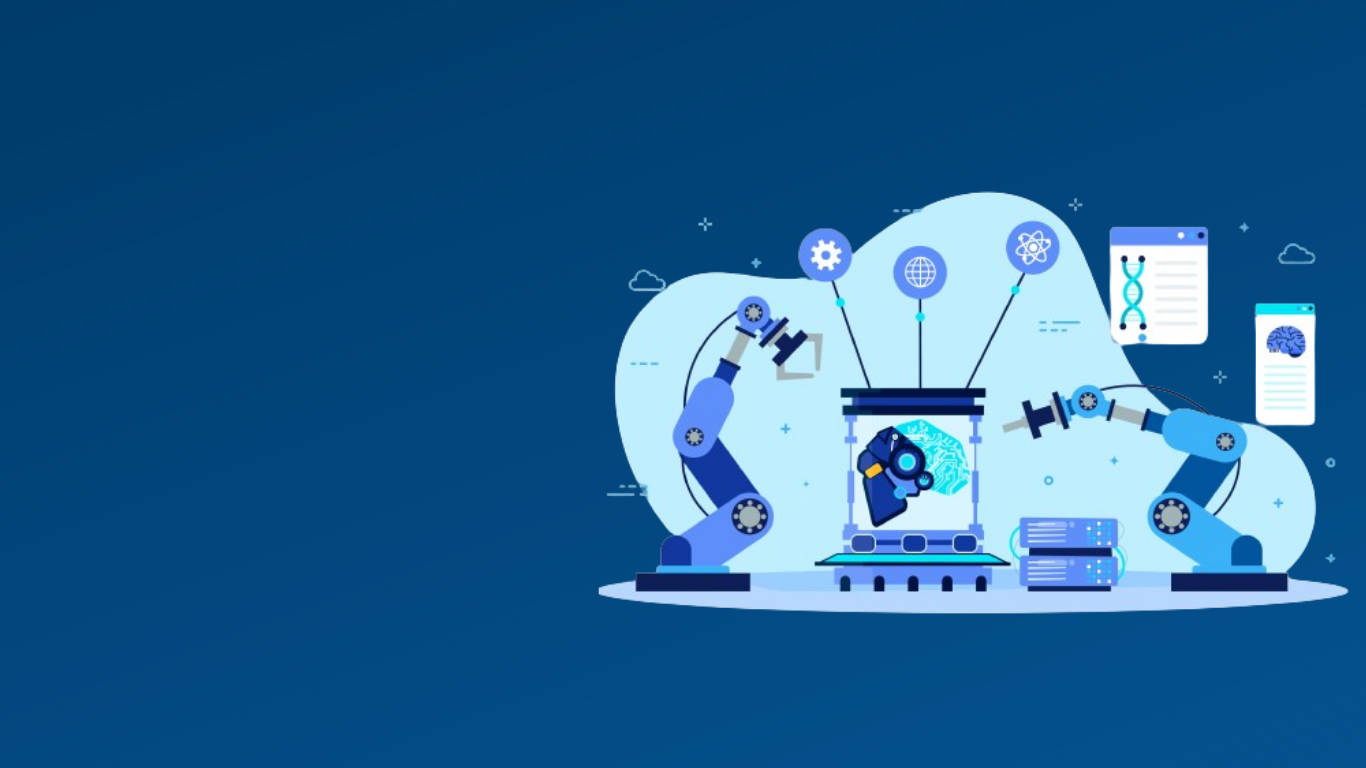
What is threat intelligence?
Threat intelligence (TI) is the strategic acquisition, processing, analysis and dissemination of information about cyber threats. It provides organizations with evidence-based knowledge on threat actors, their motivations, tools, tactics, and potential targets. Effective TI allows cybersecurity teams to make proactive risk-based decisions rather than reactive responses after threats have already impacted the business.
Challenges of Conventional Threat Intelligence
Traditional TI approaches have relied heavily on manual processes performed by human analysts and are therefore labor-intensive, slow, and lack scalability. Data is gathered from limited sources like security tool alerts, open source reports, and commercial threat feeds. Analysts then attempt to correlate and make sense of this data through manual techniques.
Overwhelming reliance on manual processes performed by human analysts
A core limitation is the overwhelming reliance on manual processes performed by human analysts. With the deluge of security data pouring in from myriad sources, analysts simply cannot keep up without automation - leading to key threats being missed or investigated too late. Additionally, these manual analysis techniques are inherently subjective and error-prone due to human biases and fatigue.
Data sources tend to be extremely limited in scope
Even when analysts can make sense of the data, the sources they analyze tend to be extremely limited in scope, potentially leading to dangerous blindspots. Most conventional programs rely only on internal security tool alerts, open source reporting, and basic commercial threat feeds. This narrow aperture fails to provide a comprehensive, contextualized view of the threat landscape that today's sophisticated actors operate in.
Fundamentally reactive and retrospective nature of traditional threat intelligence
Perhaps the most crippling limitation is the fundamentally reactive and retrospective nature of traditional threat intelligence. By the time analysts have completed their work investigating indicators of past incidents and attacks, the threats have already evolved and moved on to new vectors.
Cybersecurity teams are constantly playing catch-up rather than being proactive and anticipatory of an attacker's next move. This slow reaction cycle leaves organizations persistently exposed and unable to get ahead of threats before they strike.
Hence, some of the core limitations of TI are-
- Lack of automation results in analysts being overwhelmed by data volume
- Limited sources provide an incomplete picture with blindspots
- Manual analysis is error-prone and subjective
- Insights are delivered too slowly to keep up with rapidly evolving threats
- Resources are focused on investigating past incidents rather than predicting future threats
The Importance of Proactive Threat Intelligence in Modern Cybersecurity
Conventional reactive TI is no longer sufficient as threat actors have become more sophisticated, stealthy, and their attack cycles have accelerated.
Proactive threat intelligence empowers organizations to transition from a reactive defensive security posture to a predictive offensive stance against cyber threats. Instead of scrambling to respond after attacks occur, proactive TI provides the capability to identify emerging threats before they can impact the business.
New vulnerabilities can be discovered before attackers have a chance to exploit them. Security teams can proactively hunt for signs of threats or compromise already lurking within the environment rather than waiting for alerts to fire.
Perhaps most crucially, proactive TI enables data-driven risk-based decision making when prioritizing which threat vectors pose the highest risk and how to optimally allocate security resources. With awareness of threats looking ahead, countermeasures and mitigation techniques can be rapidly deployed preventatively rather than rushing for the latest patch after a breach.
By looking ahead at the threat landscape, proactive TI enables more effective cybersecurity control design, resource optimization, and reduced risk exposure and potential impact.
Key Components of Proactive Threat Intelligence
At the core of proactive TI capabilities are unified datasets subjected to advanced analytics techniques:
Data
- Open source data (cyber blogs, research reports, code repositories)
- Dark web monitoring for leaked data, threat actor communications
- Honeypots/honeynets for deception and threat engagement
- Crowd-sourced data from security community
- Internal data from sec tools (IDS/IPS, firewalls, proxies, etc.)
Advanced Analytics
- User & entity behavior analytics (UEBA) to detect anomalies
- Network traffic analysis to identify malicious patterns
- Natural language processing (NLP) on unstructured data sources
- Link analysis to map relationships between threats/actors
- Machine learning models for threat hunting and prediction
These advanced analytics techniques automate detection, accelerate analysis, and deliver dynamic threat intelligence by rapidly processing large, disparate data volumes, establishing baselines to identify abnormal activities, uncovering hard-to-detect patterns and subtle indicators, correlating events to stitch together the bigger picture, and forecasting potential threat evolutions and impacts.
Benefits of Proactive Threat Intelligence
By adopting a data-driven, analytics-powered proactive TI capability, organizations can realize significant business benefits:
- Earlier threat awareness and prevention of compromises: By having predictive intelligence on emerging threats, organizations can take proactive steps to prevent systems from being compromised in the first place rather than just reactively detecting after the fact.
- Faster detection of indicators of attack/compromise: Leveraging advanced analytics to automatically identify subtle indicators and patterns enables much more rapid detection of attacks or compromises already underway within the environment.
- Greater context around threats for better response: Proactive TI provides deeper context around threats like attributions, motivations, and full kill chains which allows for more informed and effective incident response procedures.
- Data-backed prioritization of vulnerability patching: Rather than blindly patching based on severity scores, analytics on real-world threat activity data allows focusing patching efforts on the vulnerabilities actually being exploited.
- Optimization of security control efficacy and costs: By understanding the highest risk threat vectors, controls can be tuned for maximum efficacy while costs are optimized by deprioritizing lower risk areas.
- Reduction in breach impacts and recovery expenses: Preventing breaches preemptively through proactive threat intelligence avoids the major operational disruptions, data loss, and recovery costs after a compromise occurs.
- Force multiplication for lean security teams: Automated analytics augments human analysts by handling data volumes and speeding analysis cycles, maximizing the impact of limited security staffing resources. Proactive TI allows organizations to make cybersecurity investments based on empirical risk assessment rather than assumptions.
Threat Intelligence Best Practices
Threat intelligence best practices provide guidelines and frameworks for organizations to effectively implement and operationalize proactive, data-driven threat intelligence capabilities. This includes methods for sourcing and processing data, structuring it for analytics, adopting the right tools, and integrating threat intelligence into security operations workflows. Some of these include-
Selecting the right sources of threat data
Effective proactive TI requires integrating multiple quality data sources across a breadth of categories like:
- Open source intelligence (OSINT)
- Dark web/darknet monitoring
- Commercial/subscribed threat feeds
- Industry/community sharing groups
- Internal security tool logs/alerts
- Threat engagements via honeypots
Variety and selective sourcing avoid blindspots and provide greater analytic context.
Determining who will acquire the data
There are a few operating models for data acquisition:
- Dedicated TI team acquires and analyzes all data
- Centralized Security Operations Center (SOC) performs TI
- Distributed with data acquired by various teams
- Managed TI service to provide data curation
The model depends on an organization's structure and available skills/resources. A hybrid approach with a centralized TI team augmented by distributed analytics is recommended.
Structuring data for analysis
Data must be properly normalized and structured through techniques like:
- Common ontologies and tagging taxonomies
- Labeling/enrichment of data fields
- Establishing consistent data formats/schemas
- Ensuring data quality and completeness
This data structuring and enrichment positions it for effective downstream advanced analytics processing.
Using tools to help with analysis
There are a variety of tools/platforms to enable proactive TI analytics:
- Security Information and Event Management (SIEM)
- User and Entity Behavior Analytics (UEBA)
- Network Traffic Analysis
- Data lakes for aggregating/storing large datasets
- Machine learning/AI model development environments
- Visualization/reporting tools for communicating insights
An integrated best-of-breed toolset for data management, analytics, automation, and operationalization is recommended.
Selecting the right tools to make data actionable
Beyond collecting and analyzing threat data, tools that operationalize the resulting intelligence are critical:
- Security Orchestration, Automation and Response (SOAR)
- Automated indicator/IOC ingestion into security enforcement points
- Closed-loop processes for validation, tuning, and updating models
- Seamless integration into existing security workflows
Maintaining tight feedback loops between operations and intelligence improves fidelity over time.
Predictive Threat Intelligence Services
For organizations lacking in-house data science capabilities or resources for a full proactive TI program, there are managed services that can provide predictive intelligence as-a-service:
- Global multi-source data collection and curation
- Advanced analytics by dedicated data science teams
- Delivery of machine-readable predictive threat intelligence
- Correlations mapped to MITRE ATT&CK framework
- Customized to customer's industry, technology stack, and risk profile
These services accelerate time-to-value for advanced proactive TI capabilities.
In a nutshell
The cyber threat landscape's increasing sophistication mandates that organizations adopt data-driven, predictive threat intelligence powered by advanced analytics. Reacting to threats is no longer sufficient - businesses need to harness big data, machine learning, and automation to proactively identify and mitigate threats before impacts occur.
By following threat intelligence best practices around data sourcing, structuring, and analytics-enablement, organizations can shift to an offensive predictive security posture. This strategic cybersecurity capability delivers a comprehensive force-multiplier effect - early threat awareness, impact/risk reduction, optimized security investments, and resilience against even the stealthiest of threat actors.
C-suite leaders should prioritize building out a robust proactive threat intelligence competency as a long-term strategic imperative for the business. The potential costs of inaction are detrimental in today's unforgiving cyber climate. To learn more on cybersecurity & compliance, read our DX Insights and gain actionable steps, strategies, roadmaps, and best practices.