Unleashing Gen AI on Edge Devices: Why Your Smartphone Might Soon Outsmart the Cloud
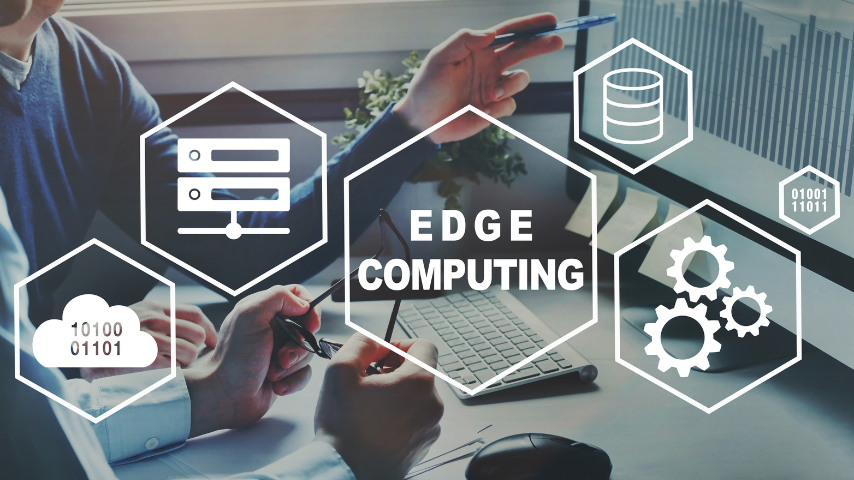
Generative AI and Edge Computing: Unleashing LLMs at the Edge
In the rapidly evolving landscape of artificial intelligence, two transformative technologies are converging to reshape the future of computing: generative AI and edge computing. As C-suite executives, understanding this intersection is crucial for staying ahead in an increasingly AI-driven world.
The Power of Generative AI Meets the Agility of Edge Computing
Generative AI, particularly Large Language Models (LLMs), has demonstrated unprecedented capabilities in natural language processing, content creation, and problem-solving. However, these models have traditionally required substantial computational resources, limiting their deployment to cloud-based infrastructures.
Enter edge computing, a paradigm that brings computation and data storage closer to the point of need. By combining generative AI with edge computing, we're on the cusp of a revolution that could democratize access to advanced AI capabilities.
The Challenge: Bringing LLMs to Resource-Constrained Devices
Deploying LLMs on edge devices presents significant challenges:
- Computational Constraints: Edge devices often have limited processing power and memory.
- Energy Efficiency: Many edge devices operate on battery power, requiring energy-efficient AI solutions.
- Model Size: LLMs can be several gigabytes in size, far exceeding the storage capacity of many edge devices.
- Real-time Performance: Edge applications often require low-latency responses, challenging for complex AI models.
Innovative Solutions: Making the Impossible Possible
Despite these challenges, innovative approaches are emerging to bring the power of LLMs to the edge:
1. Model Compression Techniques
- Quantization: Reducing the precision of model parameters without significant loss in accuracy.
- Pruning: Removing unnecessary connections in neural networks to reduce model size.
- Knowledge Distillation: Creating smaller, faster models that mimic the behavior of larger ones.
2. Specialized Hardware
- AI Accelerators: Custom chips designed for efficient AI computations on edge devices.
- Neuromorphic Computing: Brain-inspired architectures that promise higher energy efficiency for AI tasks.
3. Distributed AI Architectures
- Federated Learning: Enabling edge devices to collaboratively learn a shared model while keeping data locally.
- Split Inference: Dividing model layers between edge devices and the cloud to balance computation.
4. Adaptive AI Models
- Dynamic Neural Networks: Models that can adjust their architecture based on the available resources.
- Continual Learning: Allowing models to learn and adapt to new data on the edge device itself.
The Business Impact: Why C-Suite Executives Should Care
The convergence of generative AI and edge computing isn't just a technological marvel—it's a game-changer for businesses across industries:
- Enhanced Privacy and Security: Processing sensitive data locally reduces the risk of data breaches and complies with data regulations.
- Reduced Latency: Real-time AI responses enable new use cases in robotics, autonomous vehicles, and IoT.
- Cost Efficiency: Decreasing reliance on cloud infrastructure can significantly reduce operational costs.
- Improved Reliability: Edge AI continues to function even with unreliable network connections.
- New Market Opportunities: Enabling AI on resource-constrained devices opens up new product categories and markets.
Industry Applications: The Future is Now
The impact of edge-based generative AI is already being felt across various sectors:
- Healthcare: AI-powered diagnostic tools running on handheld devices, bringing advanced healthcare to remote areas.
- Manufacturing: Real-time quality control and predictive maintenance powered by on-device AI.
- Retail: Personalized shopping experiences delivered through AI-enabled point-of-sale systems.
- Automotive: Advanced driver assistance systems (ADAS) with on-board natural language processing.
- Smart Cities: Intelligent traffic management and public safety systems operating at the edge.
Strategies for C-Suite Executives
To capitalize on this technological convergence, consider the following strategies:
- Invest in R&D: Allocate resources to explore edge AI solutions tailored to your industry.
- Foster Partnerships: Collaborate with tech leaders and startups specializing in edge AI and hardware acceleration.
- Rethink Data Strategy: Develop a comprehensive edge data strategy that balances centralized and decentralized approaches.
- Upskill Your Workforce: Invest in training programs to build internal capabilities in edge AI development and deployment.
- Pilot Projects: Initiate small-scale edge AI projects to gain practical insights and demonstrate value.
- Prioritize Security: Implement robust security measures for edge devices running AI models.
- Stay Informed: Keep abreast of advancements in model compression and edge AI hardware.
The Road Ahead: Challenges and Opportunities
While the potential of edge-based generative AI is immense, challenges remain:
- Standardization: The lack of industry standards for edge AI could lead to fragmentation.
- Ethical Considerations: Ensuring responsible AI practices on distributed edge devices.
- Integration Complexity: Seamlessly integrating edge AI with existing cloud and on-premises infrastructure.
However, these challenges also present opportunities for forward-thinking organizations to lead and shape the future of AI at the edge.
Conclusion: Seizing the Edge AI Opportunity
The convergence of generative AI and edge computing represents a pivotal moment in the evolution of artificial intelligence. By bringing the power of LLMs to resource-constrained devices, we're unlocking new possibilities that were once thought impossible.
As C-suite executives, the time to act is now. Those who successfully navigate this technological shift will not only drive innovation within their organizations but also play a crucial role in shaping the future of computing.
The edge AI revolution is here. Are you ready to lead from the front?