Learning from the Leaders: Best Practices in AI Deployment Across Industries
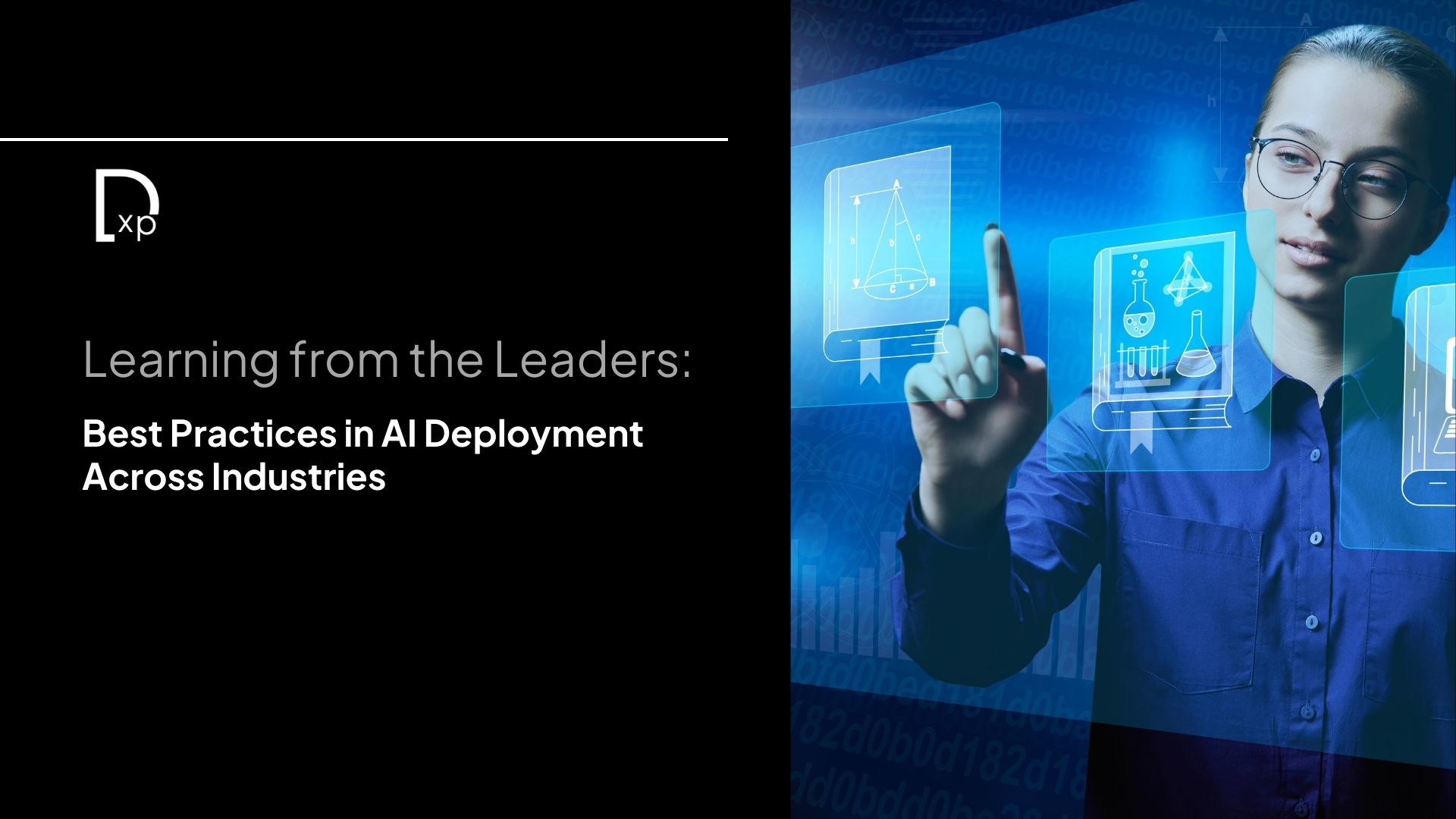
Artificial intelligence has crossed the threshold from experimental technology to foundational infrastructure. But adoption alone doesn’t guarantee success. In every industry, we’re seeing a clear divide: some organizations deploy AI with precision, purpose, and measurable results. Others struggle with stalled pilots, ethical landmines, or expensive solutions in search of a problem. The difference? Strategy. Culture. Execution. And above all, a commitment to AI best practices that go beyond theory and into action.
By learning from the leaders—those who are scaling AI responsibly and effectively—we gain insight into what it actually takes to embed intelligence into the DNA of a modern organization. From finance to healthcare, retail to manufacturing, these examples show that while AI’s form may vary, its underlying principles for success remain surprisingly consistent.
Healthcare: Augmenting, Not Replacing, the Human Touch
In healthcare, the stakes are existential. A misstep in AI deployment doesn’t just cost money—it can cost lives. That’s why the best healthcare organizations using AI aren’t trying to replace doctors, but to support them. Mayo Clinic and Cleveland Clinic, for example, have developed AI systems that assist in diagnostics, triage, and even surgical planning—yet always under human oversight.
The key best practice here is augmentation. AI is used to analyze medical images faster than radiologists can, but the final judgment rests with trained professionals. Natural language processing tools are helping to summarize doctor-patient conversations into medical records, saving hours in administrative work and improving care delivery. But none of this happens without strict compliance with HIPAA and a culture that places trust and transparency at the center.
The lesson? Deploy AI where it frees human capacity, not replaces it. Build safeguards that reinforce trust, and make explainability a non-negotiable part of the development process.
Financial Services: Automation with Accountability
The finance industry was one of the earliest adopters of AI, and it’s also among the most heavily regulated. Banks like JPMorgan Chase and Goldman Sachs are using AI to detect fraud, automate compliance reporting, and personalize wealth management at scale.
But what sets the leaders apart is their governance structure. AI models that determine creditworthiness or flag suspicious transactions are carefully audited for bias, accuracy, and fairness. They maintain tight alignment between their AI capabilities and regulatory requirements like GDPR, the Fair Credit Reporting Act, and the SEC’s emerging AI guidance.
This industry teaches us that automation isn’t enough—accountability is essential. The best practice isn’t just having powerful models, but putting the right checks, balances, and documentation in place to ensure AI supports both growth and regulatory integrity.
Retail: Hyper-Personalization Without Overstepping Privacy
Retailers are turning to AI to decode what customers want before the customers even say it. Amazon, of course, sets the gold standard with predictive analytics, dynamic pricing, and personalized product recommendations that seem almost psychic. But newer leaders like Sephora and Nike are using AI to go a step further—merging customer data with real-time feedback loops to deliver experiences that feel intuitive, not invasive.
What makes these efforts effective is their respect for data privacy. They’ve invested in zero-party and first-party data strategies, ensuring customers willingly share information in exchange for tangible value. They use AI to enhance the experience, not extract more than they should. These companies also apply AI best practices around consent, data minimization, and transparency—earning trust while scaling personalization.
The insight here is clear: AI works best when it enhances relevance, not when it crosses ethical lines. Privacy is not a tradeoff for performance—it’s a foundation for long-term brand loyalty.
Manufacturing: Predictive Maintenance and Smart Supply Chains
In manufacturing, AI isn’t glamorous—but it’s transformative. Companies like Siemens, GE, and Bosch are using machine learning to predict when equipment will fail, optimize production schedules, and streamline logistics in ways that were impossible before. These leaders are not chasing moonshots—they’re solving operational inefficiencies that drive real ROI.
Their success is rooted in the practice of continuous improvement. AI models are embedded into factory workflows, and they're retrained with new data regularly. These companies build digital twins—virtual replicas of machines and systems—where AI can test scenarios without disrupting the real-world operation.
This shows that best-in-class deployment in industrial sectors requires tight alignment between data, infrastructure, and process. The focus isn’t on innovation for innovation’s sake. It’s on measurable efficiency, reliability, and resilience.
Education and Public Sector: Responsible AI at Scale
Public institutions and education systems are turning to AI to personalize learning, streamline services, and better allocate resources. Estonia, often cited as a digital government pioneer, uses AI to deliver citizen services with unprecedented speed and precision. In education, platforms like Duolingo and Coursera are tailoring learning paths using real-time performance data, improving outcomes at scale.
What unites these efforts is responsible design. The public sector can’t afford to get AI wrong—it must be inclusive, explainable, and bias-aware. These deployments typically involve citizen feedback, public transparency, and strong ethical guidelines from the outset.
This sector reminds us that success isn’t just measured in efficiency or profit—it’s measured in trust. The best AI leaders here invite oversight, share their methodologies, and prioritize social impact alongside performance.
From Case Study to Company Culture
No matter the industry, the companies leading in AI have embraced one central truth: successful AI deployment is less about tools and more about transformation. They focus on people and processes as much as platforms. They integrate ethics, governance, and customer experience into their AI strategies from day one. They know that a good model is only as valuable as the context in which it operates.
These leaders don’t treat AI as a one-off project or a silver bullet. They treat it as a continuous evolution—one that requires experimentation, measurement, and learning. That’s the real AI best practice every organization must embrace: staying agile, staying ethical, and staying human.