Data Privacy in the Age of AI: Strategies for Compliance and Protection
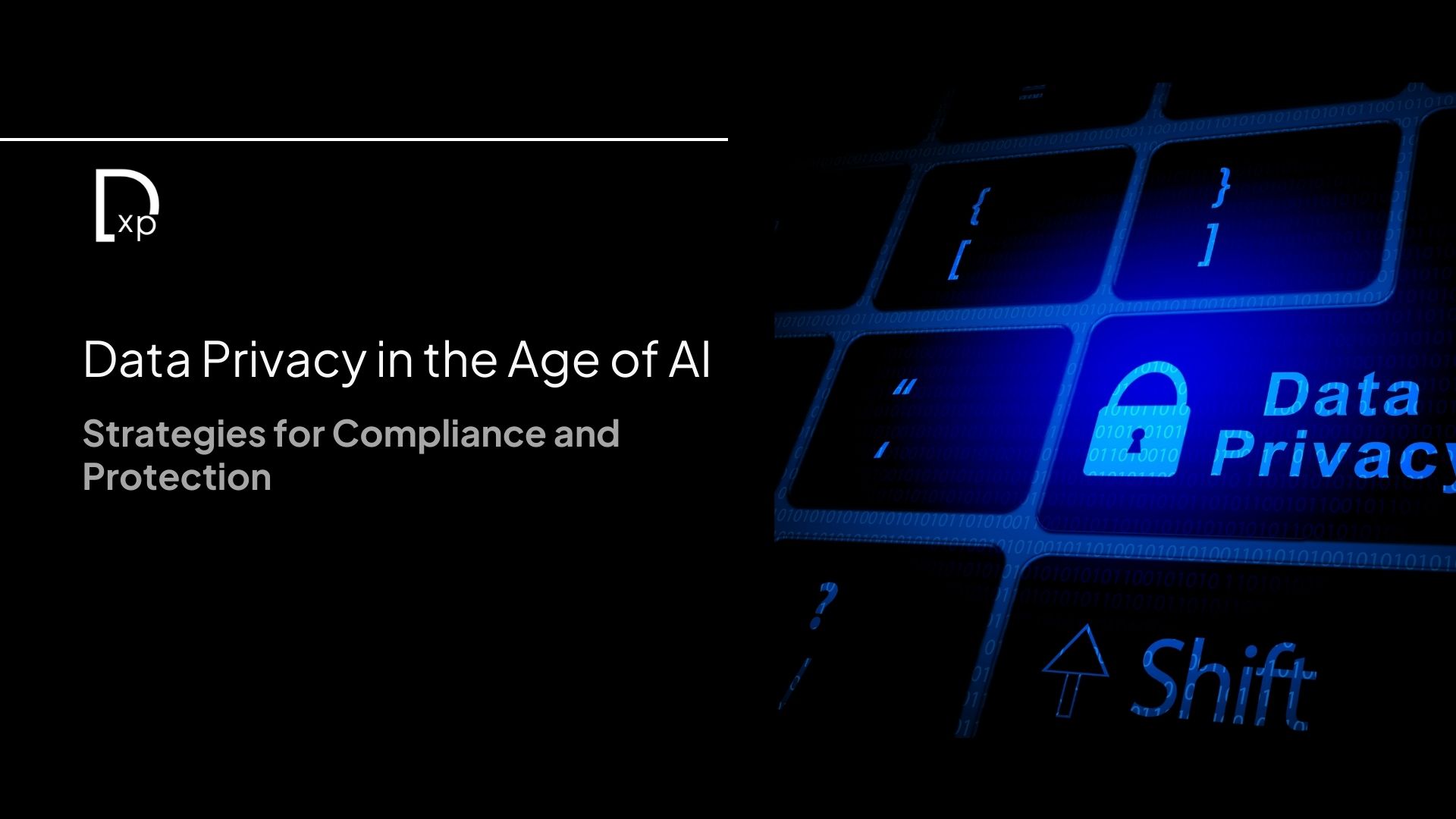
In the past decade, artificial intelligence has become the engine driving innovation across every major industry—from healthcare and finance to marketing and defense. The transformative power of AI lies in its ability to process vast volumes of data to identify patterns, predict outcomes, and make decisions at scale. But this reliance on data has simultaneously propelled privacy into the center of technological, regulatory, and ethical debate. AI and data privacy are now locked in a delicate dance, one that demands sophisticated strategies for compliance and protection.
The Data Dilemma at the Heart of AI
At the core of every powerful AI system is data—voluminous, granular, and often deeply personal. Whether it's the facial recognition systems trained on image databases, recommendation algorithms analyzing user behavior, or predictive models processing financial records, data is the lifeblood of machine learning. However, the more data an AI system consumes, the greater the potential risk to individual privacy.
This creates a profound tension: the need to unlock value from data while upholding the rights of the individuals behind it. And in a global digital economy, this tension isn’t theoretical—it’s regulated. Privacy laws such as the EU’s General Data Protection Regulation (GDPR), California’s Consumer Privacy Act (CCPA), and a growing patchwork of similar frameworks globally are forcing organizations to rethink how they collect, use, and store personal information in the context of AI.
The Challenge of Compliance in a Borderless Digital World
One of the most pressing challenges today is that while data travels seamlessly across borders, privacy regulations do not. Multinational companies must navigate a minefield of varying—and often conflicting—requirements around consent, data minimization, purpose limitation, and the right to be forgotten. These challenges are compounded when AI systems “learn” from historical data, which may no longer meet current compliance standards.
Adding to the complexity is the black-box nature of many AI systems. Even when built with compliance in mind, explaining how decisions are made can be difficult, if not impossible. This lack of transparency raises questions around accountability and fairness—issues that regulators are increasingly interested in. For example, under GDPR, individuals have the right to receive “meaningful information about the logic involved” in automated decisions. But what does that look like when even developers struggle to explain the inner workings of their neural networks?
Privacy by Design: A Strategic Imperative
To stay ahead of regulatory scrutiny and consumer expectations, companies must embed privacy into the DNA of their AI systems. This is not just a legal safeguard—it’s a strategic imperative. Privacy by design means proactively considering privacy risks from the earliest stages of development and operationalizing those principles throughout the AI lifecycle.
Techniques such as data anonymization, pseudonymization, federated learning, and differential privacy are increasingly being adopted to protect sensitive information. These technologies allow companies to train AI models without directly accessing or exposing personal data. At the same time, robust access controls, encryption standards, and audit trails ensure that data remains secure and accountable.
Yet, technology alone isn’t enough. Culture plays a critical role. Organizations must foster a mindset where ethical data stewardship is just as important as innovation. This means training engineers, data scientists, and executives alike on the nuances of data privacy and AI ethics, and building internal governance structures that can adapt as both technology and regulations evolve.
AI Ethics and the Rise of Responsible Innovation
Regulators are not the only stakeholders demanding better data practices—consumers are too. Public awareness around how personal information is used by AI systems has never been higher. Incidents involving biased algorithms, data breaches, and the misuse of personal data have eroded trust. In response, consumers are more willing than ever to walk away from companies they perceive as irresponsible.
This shift is giving rise to the concept of responsible AI—frameworks that go beyond compliance to incorporate ethical considerations such as fairness, transparency, and accountability. Companies like Microsoft, Google, and IBM have all published AI ethics principles, and many have created internal review boards to vet projects with potential ethical implications.
However, there’s still a gap between rhetoric and reality. Building AI systems that are not only compliant but also ethical requires a multidisciplinary approach, combining technical expertise with legal, philosophical, and sociological insight. As AI becomes more embedded in our daily lives, closing this gap will be crucial for long-term legitimacy and success.
Looking Ahead: A Future Built on Trust
The intersection of AI and data privacy will only grow more complex in the years ahead. As generative AI, real-time analytics, and IoT devices become more prevalent, the volume and velocity of data will skyrocket. At the same time, regulatory frameworks will continue to evolve, likely becoming stricter and more global in scope.
For businesses, the message is clear: privacy can no longer be an afterthought. It must be a foundational pillar of any AI strategy. Those who treat data privacy as a checkbox exercise will face fines, reputational damage, and loss of consumer trust. But those who approach it as an opportunity—to differentiate themselves, to innovate responsibly, and to lead by example—stand to gain not just compliance, but competitive advantage.
In the age of AI, trust is currency. And data privacy is the vault.