AI Best Practices: Ensuring Ethical Use and Maximizing ROI in Your Organization
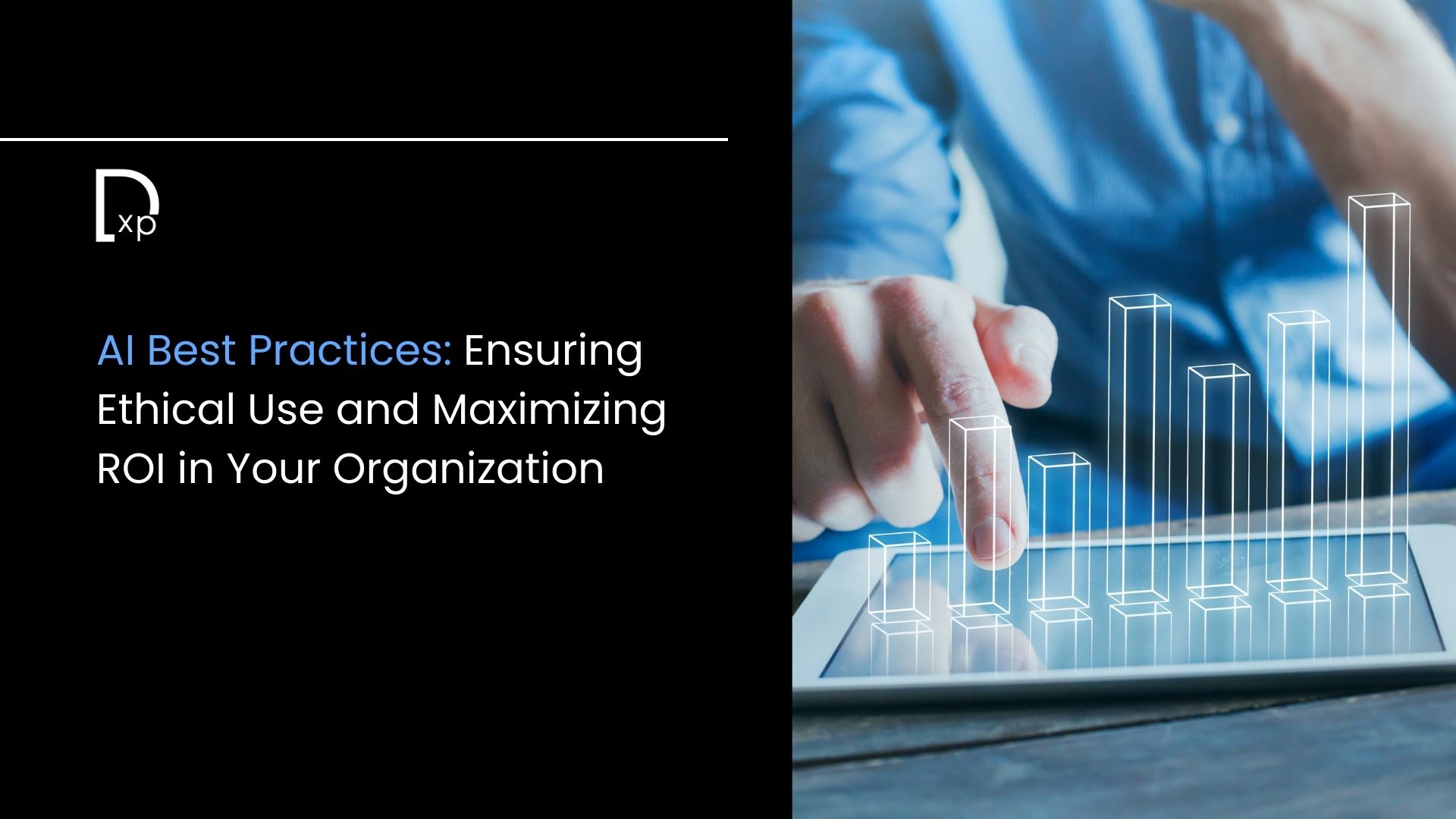
Artificial intelligence is transforming how we operate, compete, and serve. But with great power comes greater responsibility—and even greater scrutiny. For every AI breakthrough that boosts efficiency or unlocks new markets, there's a cautionary tale of bias, privacy violations, or poorly understood algorithms causing reputational harm. That's why in today’s climate, AI best practices must do double duty: ensure ethical use and drive meaningful return on investment.
The tension between ethics and performance is a false one. When done right, responsible AI is profitable AI. It builds trust with customers, reduces regulatory risk, improves internal adoption, and makes models more reliable and scalable over time. Ethics is not a constraint. It’s an enabler of long-term ROI.
Start With Purpose: Align AI With Business and Human Values
The most successful AI initiatives begin with clarity of purpose. Before any data is collected, any models are trained, or any automation is rolled out, leaders must define not only what AI is expected to achieve, but why it matters to the organization and its stakeholders.
This purpose needs to go beyond profit. It should include how AI will improve lives, solve problems, or create fairer systems. Whether it’s streamlining a customer support process, improving financial forecasting, or enhancing a healthcare diagnostic tool, tying AI to a broader mission sets the tone for ethical alignment and business relevance.
When AI is tethered to human-centric goals, it naturally encourages transparency, accountability, and fairness—factors that protect brand equity and contribute directly to ROI.
Data Discipline: The Foundation of Ethics and Accuracy
At the heart of every AI system is data—and in many ways, the ethics and effectiveness of AI are only as strong as the data it’s built on. Ethical AI demands rigorous attention to data collection, labeling, access, and usage. Biased data leads to biased outcomes. Poor-quality data leads to poor decisions. And opaque data practices lead to mistrust.
One of the critical AI best practices here is data minimization. Collect only what is necessary. Respect consent. Anonymize whenever possible. Secure data at every stage. These aren't just ethical imperatives—they’re operational necessities. Poor data hygiene leads to inefficiencies, compliance violations, and costly technical debt down the road.
At the same time, investing in a robust data governance strategy pays long-term dividends. Structured, well-maintained data improves model performance, accelerates deployment, and reduces rework—all of which contribute directly to ROI.
Build Transparency Into the Workflow
A black-box algorithm may seem impressive, but if you can’t explain how it works, you’re asking for trouble. Customers won’t trust it. Regulators won’t accept it. And your own teams won’t know when or how to intervene when things go wrong.
Explainability is one of the fastest-growing demands in AI governance, and for good reason. Leaders need to ensure that any AI deployed in high-stakes environments—such as credit scoring, hiring, healthcare, or law enforcement—has transparent decision logic. Not just for regulators, but for the humans impacted by those decisions.
That doesn’t mean every model needs to be simple. It means that the decision-making process must be understandable, documented, and open to scrutiny. Embedding transparency into development also improves internal adoption. Teams are more likely to embrace AI tools they can trust—and tools they trust deliver more consistent value.
Create Ethical Guardrails, Not Just Guidelines
A PowerPoint on AI ethics won’t prevent a crisis. Culture, processes, and incentives must be designed to support ethical behavior at every step of AI development and deployment. That starts with governance structures.
Who’s responsible for reviewing AI projects? Are there escalation paths for ethical concerns? Is bias testing part of the standard workflow? Do you conduct impact assessments before launch? Are there defined criteria for when to retrain or decommission a model?
The most mature organizations are building cross-functional AI ethics boards, embedding bias monitors into their CI/CD pipelines, and establishing internal red-teaming practices to anticipate unintended consequences. This kind of infrastructure doesn’t slow innovation—it de-risks it. It catches problems early and ensures your AI initiatives remain aligned with both mission and market.
Measure ROI Holistically
Maximizing ROI from AI means redefining what return really means. Yes, automation can reduce headcount or speed up processes. But some of the highest-value gains come from less tangible, but equally powerful, outcomes: better customer retention, faster decision-making, improved employee satisfaction, stronger compliance posture.
That’s why AI best practices include building ROI models that look beyond traditional KPIs. Track time saved, error rates reduced, insights gained, and customer sentiment improved. Analyze how AI supports strategic goals like scalability, personalization, and innovation readiness.
Also consider the cost of not doing it right: fines, reputational damage, missed market opportunities, and disengaged teams. The return on ethical AI isn’t always obvious on a quarterly report—but it compounds over time.
Train Your People—Not Just Your Models
AI transformation fails not because the technology doesn’t work, but because the people don’t trust it or don’t understand it. No matter how advanced your models, if employees don’t know how to use them—or worse, don’t want to—they will sit unused.
One of the most undervalued AI best practices is education. Train your people across departments—not just technical teams—on what AI is, what it can do, and how it fits into their roles. Demystify it. Build fluency. Create a culture where AI isn’t feared or misunderstood, but embraced as a tool to empower.
This internal trust accelerates adoption, improves decision-making, and fosters a mindset of continuous learning—all of which improve your ability to extract ROI from AI over the long term.
Conclusion: Responsible AI Is High-Performance AI
It’s no longer acceptable—or profitable—to treat ethics and performance as competing priorities in AI deployment. The organizations that win with AI are not just the ones that move fast, but the ones that move smart. They build ethical frameworks not as an afterthought, but as part of their operational DNA. They treat data with care. They demand clarity from their algorithms. And they invest in both technology and people.
The bottom line is this: if you want AI that works, scales, and lasts—you need AI that earns trust. Because in the age of intelligence, responsibility is the real accelerator of results.