Machine Learning Unlocks New Cryoprotectants for Safer Cold Storage
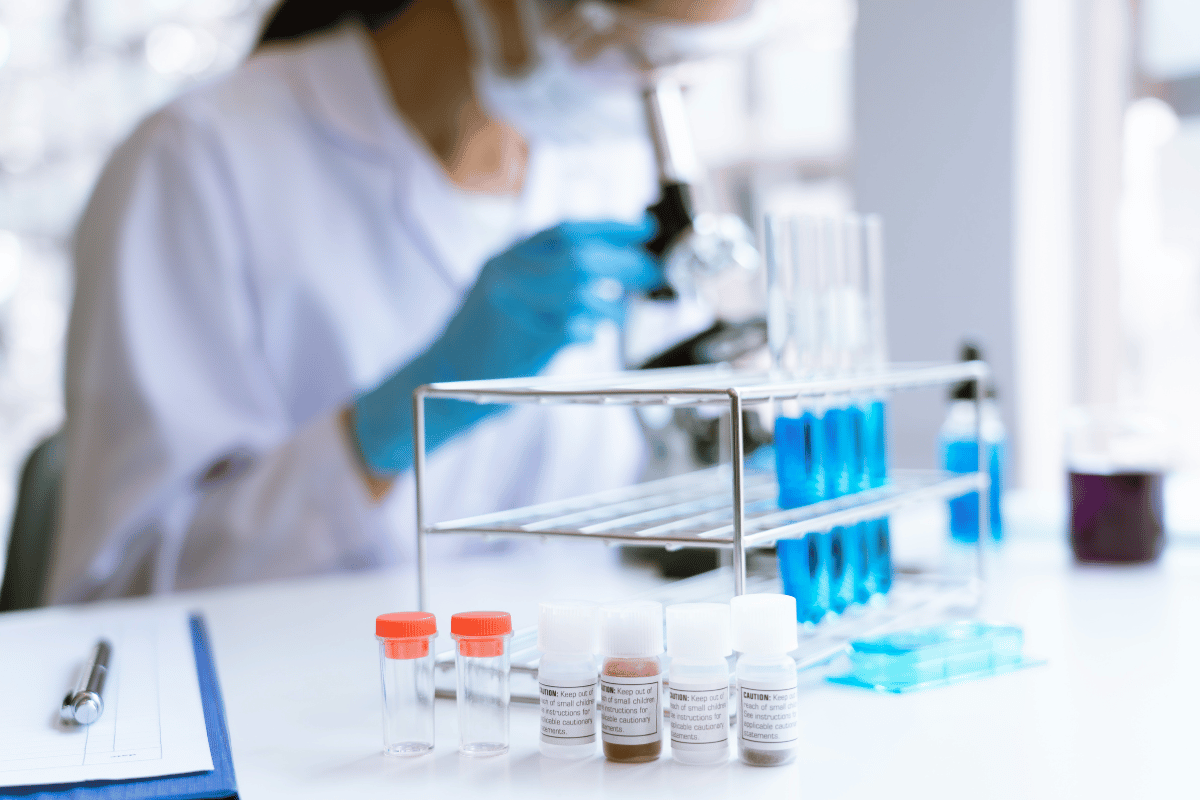
In a ground-breaking finding, researchers from the Universities of Warwick and Manchester have revolutionized the discovery of cryoprotectants, which are critical chemicals for protecting treatments, such as vaccines, fertility materials, and blood donations, using machine learning. This new computational framework enables hundreds of molecules to be tested virtually, vastly improving the efficiency of cryoprotectant discovery compared to traditional trial-and-error methods.
The research, published in Nature Communications, introduces a machine learning model capable of predicting which molecules can best prevent ice crystal formation during freezing. Ice crystals pose a significant challenge to cryopreservation, as they can damage cells during both freezing and thawing. Current cryoprotectants can protect cells but often fail to stop ice crystal growth, limiting their effectiveness.
The use of machine learning in this research marks a significant shift in how scientists approach the discovery of new cryoprotectants. By analyzing vast libraries of chemical compounds, the model identifies which molecules are most likely to be effective, speeding up the process and reducing the time spent on labor-intensive experiments. This data-driven approach allows researchers to focus on more complex challenges that still require human expertise, while routine tasks are automated.
This breakthrough has far-reaching implications. By reducing the amount of traditional cryoprotectant required for blood storage, the newly discovered molecules could allow blood to be transfused more quickly, speeding up critical medical processes. The ability to test and discover cryoprotectants faster will accelerate advancements in medical treatments and vaccines, ensuring that these critical resources are more widely available and effective.
Machine learning’s growing role in scientific research is evident in this project’s success. By combining AI with molecular simulations and experimental data, the research team demonstrated how technology can accelerate scientific discovery and solve complex challenges that would have been impossible to tackle manually. This achievement is a clear example of how machine learning is shaping the future of scientific research.
As cryopreservation becomes increasingly important for the long-term storage of medicines and biological materials, this new computational approach could be a game-changer for the field. The collaboration between Prof. Gabriele Sosso and Prof. Matthew Gibson highlights how integrating AI into traditional scientific processes can lead to revolutionary outcomes. The model identified molecules that seasoned experts might never have chosen, showcasing the power of machine learning in expanding scientific possibilities.
The findings could also lead to the repurposing of existing molecules known to slow or stop ice crystal growth, further accelerating the discovery of novel cryoprotectants. This approach offers a cost-effective and time-saving solution to a problem that has long plagued cryogenic storage.
As AI continues to evolve, its ability to handle vast amounts of data and identify patterns that humans may overlook will push the boundaries of what is possible in fields like medicine, biology, and materials science. This breakthrough is a clear sign that machine learning is not just a tool of convenience but a critical driver of innovation.
Want to stay updated on the latest tech breakthroughs? Follow our tech news to discover more advancements shaping the future of science and technology.