Why Poor Data Quality Could Be Costing You Millions
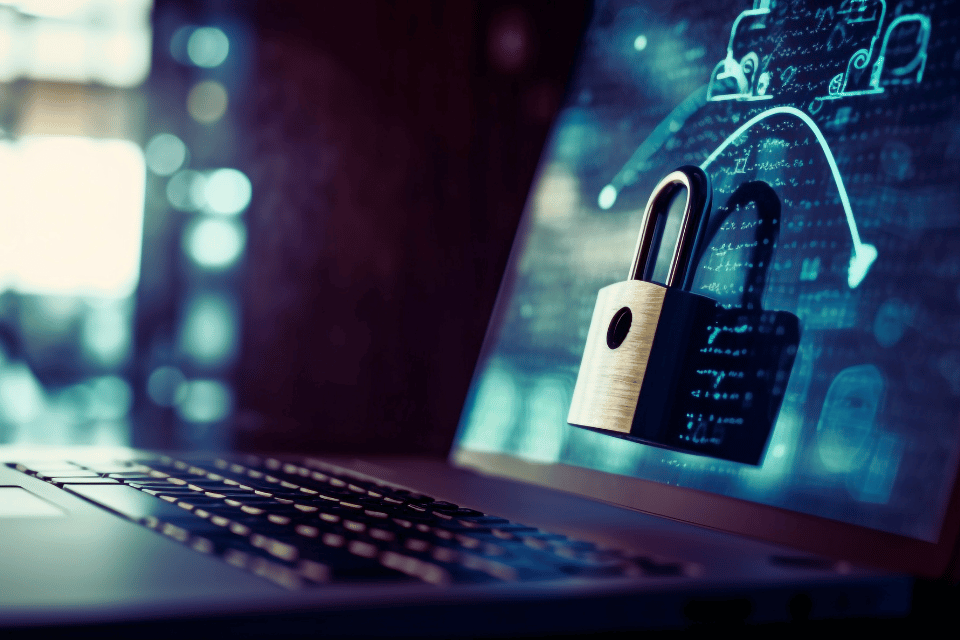
Unlocking the True Potential of Data
In today’s data-driven landscape, the importance of high-quality data cannot be overstated. According to Gartner, poor data quality costs organizations an average of $12.9 million annually—a staggering figure that should serve as a wake-up call for any enterprise aiming to leverage data for competitive advantage. As the role of data and analytics (D&A) continues to grow, the consequences of neglecting data quality are becoming increasingly dire, especially for C-suite executives who are responsible for steering their organizations through digital transformation.
The Hidden Cost of Poor Data Quality
Data quality is more than a technical issue; it's a business imperative. When data is inaccurate, incomplete, or inconsistent, it leads to poor decision-making, wasted resources, and missed opportunities. The regulatory landscape, with laws like GDPR and CCPA, further complicates the matter by imposing stringent requirements on how organizations manage and protect data. This adds another layer of complexity—and cost—to the equation.
Why Data Quality is a Business Issue
Data quality often gets sidelined, treated as a problem for the IT department rather than a business-wide concern. However, Gartner’s insights make it clear that data quality is a business discipline that requires ownership and collaboration across departments. The lack of ownership is a critical barrier, with many business leaders failing to see how data in their domain impacts broader enterprise outcomes.
For example, inconsistent data across multiple sources is one of the most challenging issues to address. This inconsistency often results from data being stored in silos, making standardization difficult. In an era where AI and machine learning are becoming integral to business strategy, the inability to connect and standardize data can severely limit the effectiveness of these technologies.
Prioritizing Data Quality for Maximum Impact
Not all data is created equal, and neither should your data quality efforts be. Gartner recommends setting the scope of your data quality program based on the organization’s most important business use cases. By mapping these use cases along dimensions of value and risk, organizations can prioritize efforts where high-quality data will deliver the most significant benefits or where poor data quality poses the greatest risks.
For instance, centralized data—such as master data that is critical and widely used—should have a broad scope of data quality efforts, involving multiple stakeholders. On the other hand, local data that serves a single purpose may require less rigorous oversight.
Measuring What Matters: Data Quality Metrics
One of the most alarming findings from Gartner is that 59% of organizations do not measure data quality. This oversight makes it difficult to quantify the impact of poor data quality or track improvements over time. To tackle this, organizations must first understand the dimensions of data quality—such as accuracy, completeness, and timeliness—and then choose the metrics that are most relevant to their key use cases.
Once the right metrics are in place, data profiling can be used to assess the current state of data quality. This involves both column-based profiling, which provides statistical information about data, and rule-based profiling, which validates data against business logic. The insights gained from profiling can then be visualized in a heat map, making it easier to identify and prioritize data quality issues.
The Future of Data Quality: AI and Augmented Solutions
As organizations look to the future, the role of AI and augmented data quality solutions cannot be ignored. These technologies represent a fundamental shift in how data quality issues are addressed, using active metadata, natural language processing (NLP), and graph technologies to accelerate processes and reduce risk.
Gartner identifies 10 critical capabilities that organizations should consider when evaluating data quality solutions, including profiling, parsing, and standardization, as well as advanced features like automation and augmentation. By leveraging these modern tools, organizations can not only improve data quality but also enhance the overall value of their data and analytics programs.
Conclusion: A Call to Action for C-Suite Executives
For C-suite executives, the message is clear: data quality is not just an operational concern but a strategic one that impacts the entire organization. By prioritizing data quality based on key business use cases, measuring what matters, and leveraging the latest AI-driven solutions, executives can unlock the true potential of their data, driving better decision-making and delivering a significant return on investment.
In a world where data is the new oil, the quality of that data will determine whether your organization thrives or falls behind. Don't let poor data quality cost you millions—take action now to ensure your data is a valuable asset rather than a liability.