Generative AI Architecture: A Deep Dive for C-Suite Executives
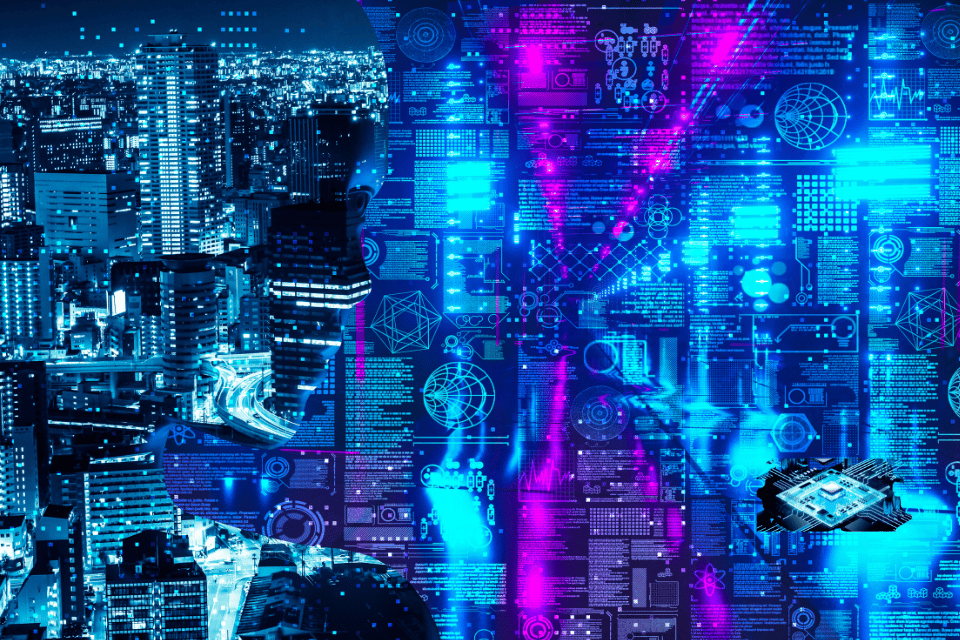
Generative AI has emerged as a transformative force in numerous industries, capable of creating new content, designs, and even complex strategies through advanced algorithms. At the heart of this capability lies the architecture of generative AI, which is both intricate and powerful. Understanding this architecture is crucial for C-suite executives who aim to leverage AI to drive innovation, efficiency, and competitive advantage within their organizations. This blog provides a comprehensive exploration of generative AI architecture, offering insights into its components, working mechanisms, and strategic implications.
Understanding the Core Components of Generative AI Architecture
Generative AI architecture comprises several key components that work together to produce new content or data. These components include neural networks, transformers, generative adversarial networks (GANs), variational autoencoders (VAEs), and reinforcement learning. Each plays a distinct role in enabling generative AI to function effectively.
1. Neural Networks: At the foundation of generative AI lies the neural network, a system modeled after the human brain’s structure. Neural networks consist of layers of nodes (or neurons) that process input data and produce outputs. These networks learn from large datasets, identifying patterns and making predictions. In generative AI, neural networks are trained to create new content based on learned patterns, such as generating text, images, or even music.
For example, OpenAI’s GPT-3 (Generative Pre-trained Transformer 3) is a neural network-based model that can generate human-like text. GPT-3’s architecture involves 175 billion parameters, making it one of the most advanced models of its kind. It can write essays, generate code, and even create poetry, demonstrating the power of neural networks in generative AI.
2. Transformers: Natural language processing (NLP) has been transformed by a class of model designs known as transformers. Introduced in 2017, transformers use self-attention mechanisms to process input data and generate output sequences. They are particularly effective in tasks involving sequence-to-sequence transformations, such as translation and summarization.
Transformers are the backbone of models like GPT-3 and BERT (Bidirectional Encoder Representations from Transformers). They excel at handling large datasets and can generate coherent and contextually relevant content. For businesses, transformers enable applications such as chatbots, content creation tools, and automated translation services.
3. Generative Adversarial Networks (GANs): Both a generator and a discriminator are two neural networks that operate in tandem to form GANs. The generator generates new data, which the discriminator compares to real data. To "fool" the discriminator, the generator must create content that is identical to actual data.
GANs are widely used in generating realistic images, videos, and even audio. For instance, GANs have been employed to create deepfakes, where AI-generated content mimics real people’s appearances and voices. While deepfakes raise ethical concerns, the underlying technology has legitimate applications in marketing, entertainment, and virtual reality.
4. Variational Autoencoders (VAEs): VAEs are a type of autoencoder that is particularly useful for generating new data points in a continuous space. Unlike traditional autoencoders, which compress data into a fixed-size latent space, VAEs introduce variability, allowing for the generation of diverse outputs.
VAEs are commonly used in generating images, 3D models, and other complex data types. They are also valuable in tasks that require exploring the latent space of data, such as drug discovery and material design. For example, in the pharmaceutical industry, VAEs can generate new molecular structures with desired properties, accelerating the drug development process.
5. Reinforcement Learning: It is a kind of machine learning where an agent learns to make decisions through interaction with its surroundings. The agent receives rewards or penalties based on its actions, and over time, it learns to optimize its behavior to maximize cumulative rewards.
In generative AI, reinforcement learning is often used in combination with other models to improve performance. For example, reinforcement learning can be used to fine-tune a generative model by rewarding it for producing high-quality outputs or penalizing it for generating errors. This approach is particularly useful in applications like game design, where AI can generate new levels or scenarios that are both challenging and engaging.
How Generative AI Architecture Drives Business Innovation
The architecture of generative AI is not just a technical marvel; it is a powerful tool for driving business innovation. By leveraging the capabilities of generative AI, businesses can unlock new opportunities, improve efficiency, and gain a competitive edge in their industries.
1. Content Creation and Automation: Content generation is one of the most practical applications of generative AI. Businesses can use AI to generate marketing copy, product descriptions, and even entire articles. This not only saves time and resources but also allows for the creation of personalized content at scale.
For example, a retail company could use generative AI to create personalized product descriptions based on customer preferences. This level of personalization can enhance the customer experience and increase conversion rates.
2. Product Design and Development: Generative AI can also be used in product design and development. By analyzing existing designs and user feedback, AI can generate new product concepts that meet customer needs more effectively. Businesses can develop innovative products more quickly and launch them to the market with this strategy.
In the automotive industry, for instance, generative AI can be used to design more efficient engines or optimize vehicle aerodynamics. By simulating various design options, AI can identify the best-performing configurations, reducing the need for physical prototypes and accelerating the development process.
3. Decision Support and Strategy: Generative AI can provide valuable insights that support decision-making at the executive level. By analyzing vast amounts of data, AI can generate scenarios, forecasts, and recommendations that help executives make informed decisions.
For example, a financial services firm might use generative AI to generate different market scenarios based on economic indicators. These scenarios can help the firm anticipate potential risks and opportunities, allowing it to adjust its strategies accordingly.
4. Enhanced Customer Experiences: Generative AI is also transforming customer interactions. AI-powered chatbots and virtual assistants can provide personalized support, answering customer queries, and resolving issues in real-time. This lowers the workload for human support staff and boosts customer satisfaction.
In the hospitality industry, for example, a hotel chain could use generative AI to provide personalized recommendations for guests, such as suggesting local attractions based on their interests. This enhances the guest experience and fosters customer loyalty.
The Future of Generative AI Architecture
As we look ahead, the future of generative AI architecture is set to be defined by several transformative trends and innovations that will push the boundaries of what AI can achieve in business and beyond. Here’s a fresh perspective on what to expect:
1. Hyper-Personalization at Scale: The next generation of generative AI will enable hyper-personalization on a massive scale. Businesses will be able to create highly tailored experiences for individual customers, driven by AI models that analyze and generate content based on real-time data. This level of personalization will extend beyond marketing and customer service to areas like product development and supply chain management, where AI can tailor solutions to specific customer needs and preferences.
2. Collaborative AI Ecosystems: The future of generative AI architecture will likely involve more collaborative ecosystems where multiple AI models interact and learn from each other. These AI ecosystems will allow for more complex problem-solving and innovation by combining the strengths of different models, such as integrating NLP with visual recognition AI or combining generative design with predictive analytics. This collaborative approach will enable businesses to tackle multifaceted challenges with greater efficiency and creativity.
3. AI-Driven Innovation in Emerging Markets: As generative AI becomes more accessible, its architecture will play a crucial role in driving innovation in emerging markets. In regions where technological infrastructure is still developing, AI could leapfrog traditional development paths, enabling businesses to innovate rapidly and address local challenges with AI-driven solutions. For instance, in healthcare, generative AI could help create affordable and tailored medical solutions that cater to specific regional needs.
4. Autonomous AI Systems: The evolution of generative AI will likely lead to more autonomous systems capable of making decisions and taking actions with minimal human intervention. These systems will be built on architectures that enable continuous learning and adaptation, allowing them to operate effectively in dynamic and unpredictable environments. Autonomous AI could revolutionize industries like logistics, where AI-driven systems manage entire supply chains with minimal human oversight, optimizing operations in real-time.
5. Democratization of AI Development: As generative AI architectures become more advanced, they will also become more user-friendly, enabling non-experts to build and deploy AI models. This democratization of AI development will empower more businesses, especially small and medium-sized enterprises (SMEs), to leverage AI for competitive advantage. Low-code and no-code AI platforms will be at the forefront of this trend, allowing users to create AI solutions without needing deep technical expertise.
6. Sustainable and Green AI: The future of generative AI architecture will also prioritize sustainability. As AI models grow in complexity and computational demands, there will be a push towards developing energy-efficient AI systems that minimize environmental impact. This includes optimizing AI algorithms for lower power consumption and developing AI hardware that is more environmentally friendly. Businesses will need to balance the benefits of AI-driven innovation with the imperative of reducing their carbon footprint.
7. Ethical AI and Transparency: As generative AI becomes more pervasive, ethical considerations will become increasingly important. Businesses will need to ensure that their AI systems are transparent, fair, and free from bias. This will require ongoing monitoring and evaluation of AI models, as well as the development of ethical guidelines and standards.
8. AI-Augmented Creativity: The future of generative AI also holds the promise of AI-augmented creativity. By collaborating with AI, human creators can push the boundaries of what is possible, creating new forms of art, music, and design that were previously unimaginable. For example, in the fashion industry, AI could assist designers in creating innovative clothing designs by generating new patterns and styles based on current trends. This collaboration between human creativity and AI capabilities could lead to a new era of artistic expression.
Is your organization prepared to lead in the era of next-generation AI?
Read our latest blog on Generative AI in Competitive Intelligence. Explore our other insightful blogs on our website to discover how we can help you build sustainable, scalable, and impactful AI solutions tailored to your strategic goals.